Multi-Task Transformer Visualization to build Trust for Clinical Outcome Prediction
Dario Antweiler, Florian Gallusser, Georg Fuchs
Room: 104
2023-10-22T03:00:00ZGMT-0600Change your timezone on the schedule page
2023-10-22T03:00:00Z
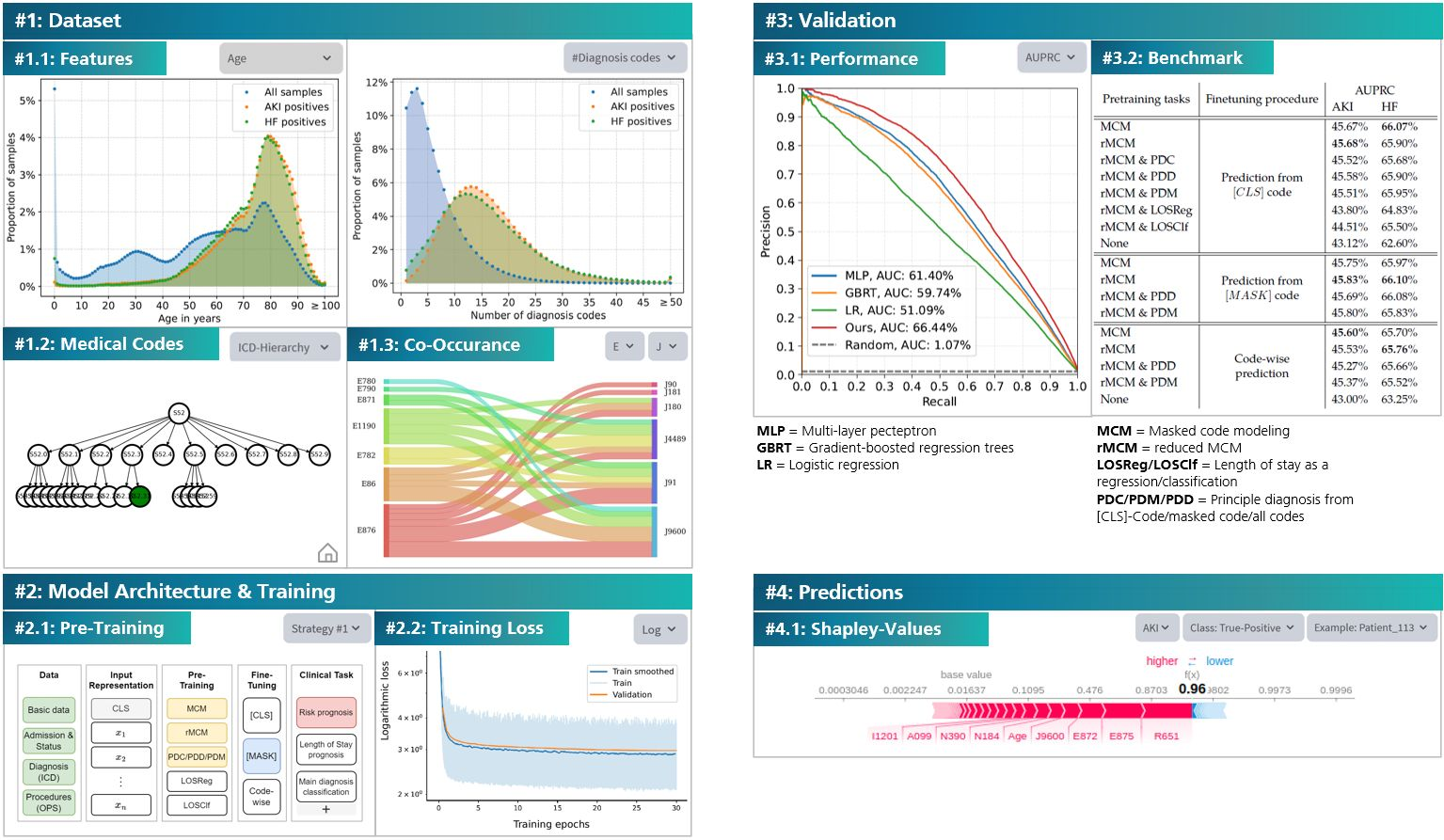
Fast forward
Full Video
Keywords
Outcome prediction, Explainable AI and ML in healthcare, Clinical workflows, Patient safety
Abstract
Clinical decision support systems based on machine learning are a rising application in healthcare. Early detection of deteriorating conditions provide the opportunity for medical intervention in hospital patients. Recent approaches increasingly rely on Large Language Models such as BERT, because patient data is often in the form of structured temporal data. These models are notoriously hard to interpret and therefore to trust, while precisely trust is an essential principle for technology in healthcare. We develop a visual analytics system to inspect, compare, and explain pre-trained transformer models for a given clinical outcome prediction task. The work is developed on the basis of a large hospital patient dataset and prediction tasks for acute kidney injury and heart failure. Discussion with healthcare professionals confirms that our system can lead to a faster decision process and improved modeling results.