ExpLIMEable: A Visual Analytics Approach for Exploring LIME
Sonia Laguna, Julian Heidenreich, Jiugeng Sun, Nilüfer Çetin, Ibrahim Al-Hazwani, Udo Schlegel, Furui Cheng, Mennatallah El-Assady
Room: 104
2023-10-22T03:00:00ZGMT-0600Change your timezone on the schedule page
2023-10-22T03:00:00Z
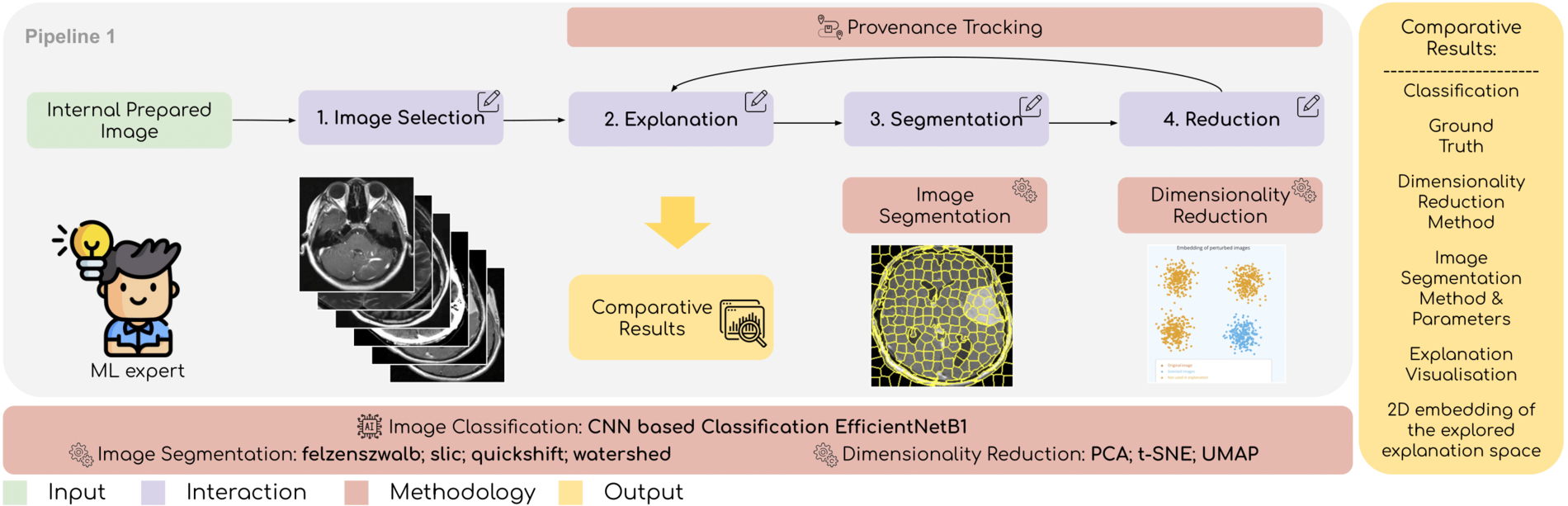
Keywords
Explainable AI Visualization LIME Healthcare
Abstract
We introduce ExpLIMEable for enhancing the understanding of Local Interpretable Model-Agnostic Explanations (LIME), with a focus on medical image analysis. LIME is a popular and widely used method in explainable artificial intelligence (XAI) that provides locally faithful and interpretable post-hoc explanations for black box models. However, LIME explanations are not always robust due to variations in perturbation techniques and the selection of interpretable functions. The proposed visual analytics application aims to address these concerns by enabling the users to freely explore and compare the explanations generated by different LIME parameter instances. The application utilizes a convolutional neural network (CNN) for brain MRI tumor classification and allows users to customize post-hoc LIME parameters to gain insights into the model’s decision-making process. The developed application assists machine learning developers in understanding the limitations of LIME and its sensitivity to different parameters, as well as the doctors in providing an explanation to machine learning models, enabling more informed decision-making, with the ultimate goal of improving its robustness and explanation quality.