Temporal Merge Tree Maps: A Topology-Based Static Visualization for Temporal Scalar Data
Wiebke Köpp, Tino Weinkauf
View presentation:2022-10-20T20:45:00ZGMT-0600Change your timezone on the schedule page
2022-10-20T20:45:00Z
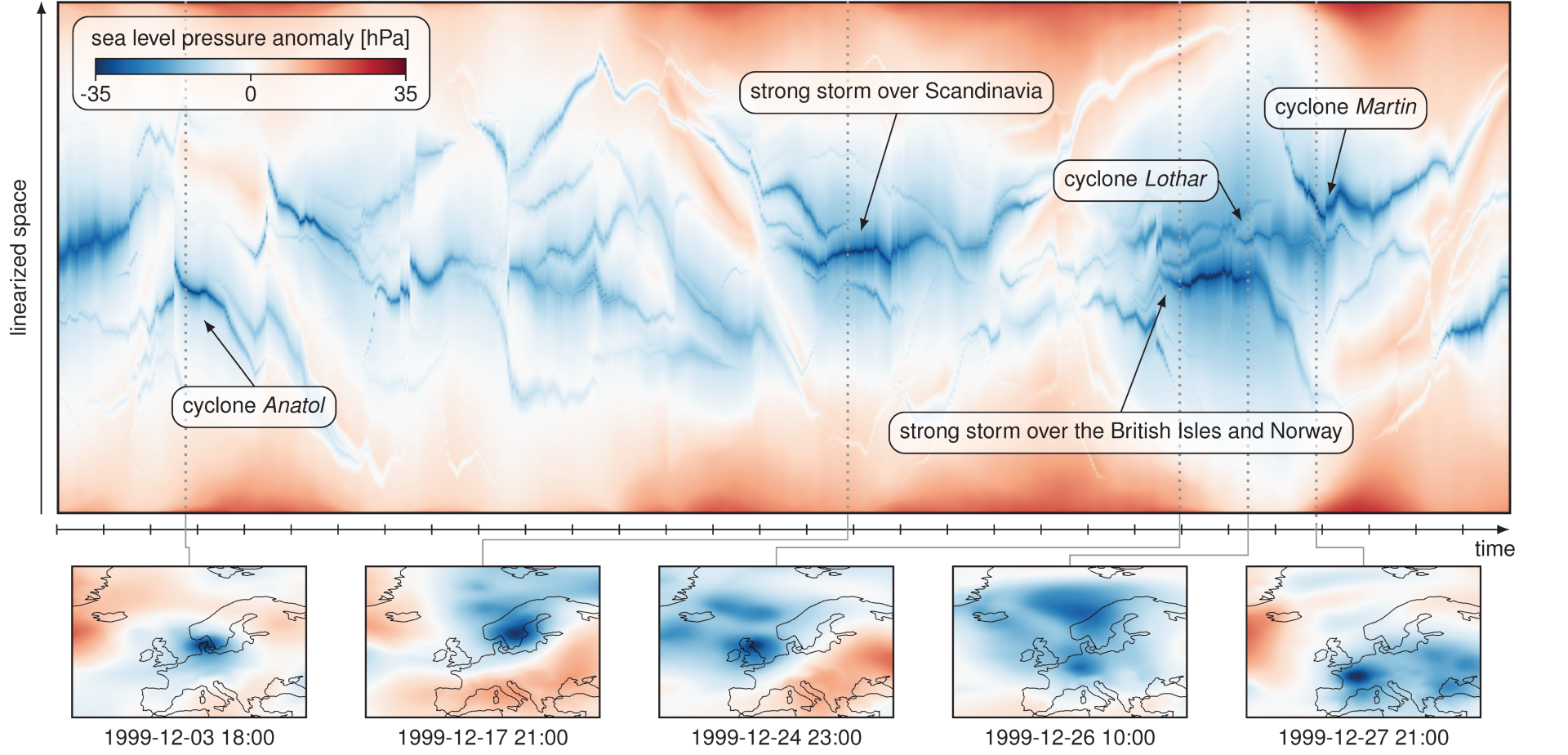
Prerecorded Talk
The live footage of the talk, including the Q&A, can be viewed on the session page, Topology.
Fast forward
Abstract
Creating a static visualization for a time-dependent scalar field is a non-trivial task, yet very insightful as it shows the dynamics in one picture. Existing approaches are based on a linearization of the domain or on feature tracking. Domain linearizations use space-filling curves to place all sample points into a 1D domain, thereby breaking up individual features. Feature tracking methods explicitly respect feature continuity in space and time, but generally neglect the data context in which those features live. We present a feature-based linearization of the spatial domain that keeps features together and preserves their context by involving all data samples. We use augmented merge trees to linearize the domain and show that our linearized function has the same merge tree as the original data. A greedy optimization scheme aligns the trees over time providing temporal continuity. This leads to a static 2D visualization with one temporal dimension, and all spatial dimensions compressed into one. We compare our method against other domain linearizations as well as feature-tracking approaches, and apply it to several real-world data sets.