Uncertainty-Aware Multidimensional Scaling
David Hägele, Tim Krake, Daniel Weiskopf
View presentation:2022-10-18T16:30:00ZGMT-0600Change your timezone on the schedule page
2022-10-18T16:30:00Z
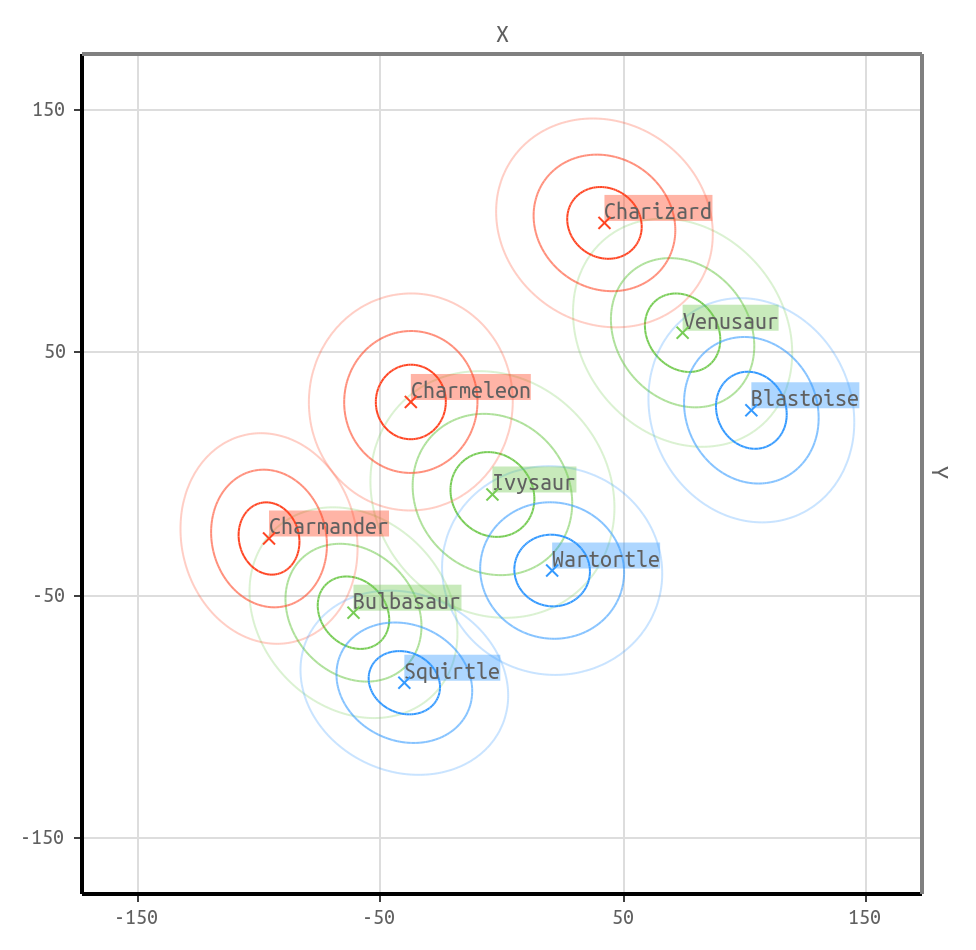
Prerecorded Talk
The live footage of the talk, including the Q&A, can be viewed on the session page, VIS Opening (10:45am-11:03am)| Best Papers (11:03am-12:00pm).
Fast forward
Abstract
We present an extension of multidimensional scaling (MDS) to uncertain data, facilitating uncertainty visualization of multidimensional data. Our approach uses local projection operators that map high-dimensional random vectors to low-dimensional space to formulate a generalized stress. In this way, our generic model supports arbitrary distributions and various stress types. We use our uncertainty-aware multidimensional scaling (UAMDS) concept to derive a formulation for the case of normally distributed random vectors and a squared stress. The resulting minimization problem is numerically solved via gradient descent. We complement UAMDS by additional visualization techniques that address the sensitivity and trustworthiness of dimensionality reduction under uncertainty. With several examples, we demonstrate the usefulness of our approach and the importance of uncertainty-aware techniques.