Development and Evaluation of Two Approaches of Visual Sensitivity Analysis to Support Epidemiological Modeling
Erik Rydow, Rita Borgo, Hui Fang, Thomas Torsney-Weir, Ben Swallow, Thibaud Porphyre, Cagatay Turkay, Min Chen
View presentation:2022-10-21T14:36:00ZGMT-0600Change your timezone on the schedule page
2022-10-21T14:36:00Z
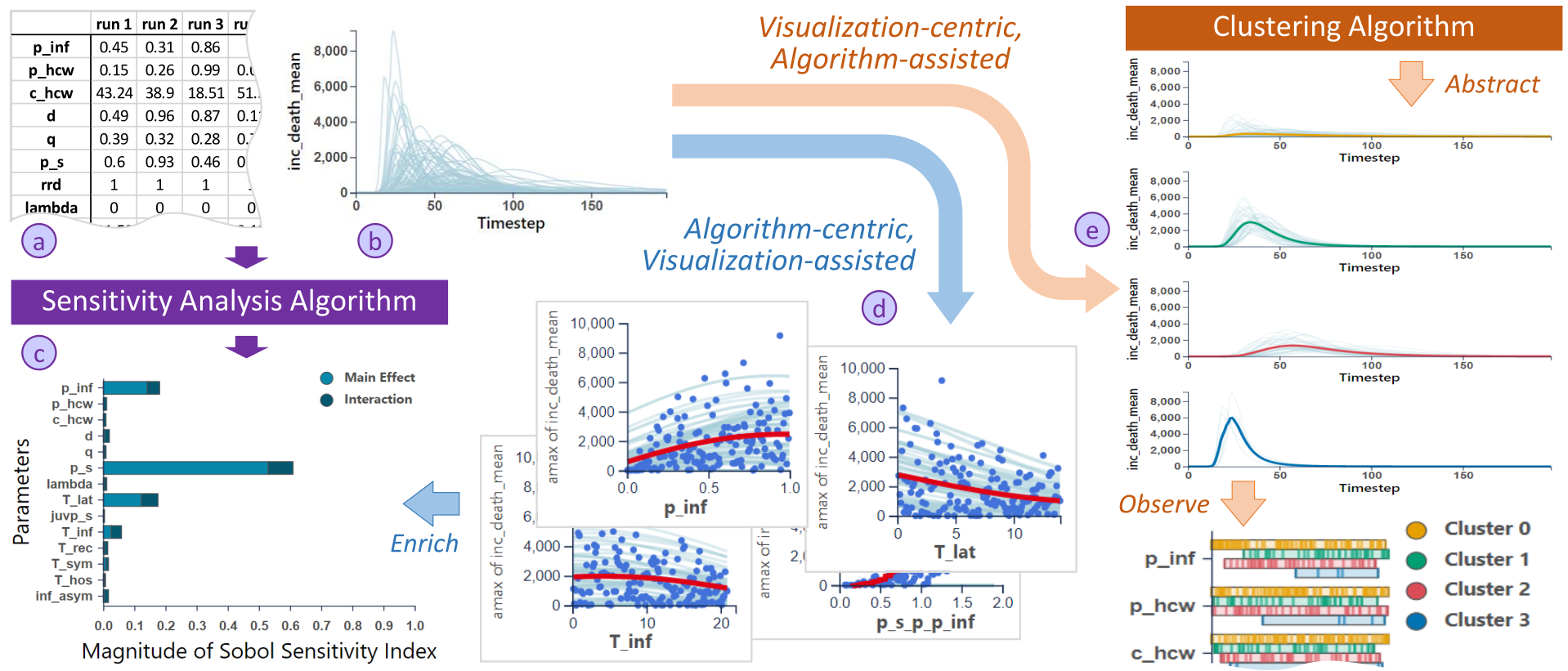
Prerecorded Talk
The live footage of the talk, including the Q&A, can be viewed on the session page, Visual Analytics of Health Data.
Fast forward
Abstract
Computational modeling is a commonly used technology in many scientific disciplines and has played a noticeable role in combating the COVID-19 pandemic. Modeling scientists conduct sensitivity analysis frequently to observe and monitor the behavior of a model during its development and deployment. The traditional algorithmic ranking of sensitivity of different parameters usually does not provide modeling scientists with sufficient information to understand the interactions between different parameters and model outputs, while modeling scientists need to observe a large number of model runs in order to gain actionable information for parameter optimization. To address the above challenge, we developed and compared two visual analytics approaches, namely: algorithm-centric and visualization-assisted, and visualization-centric and algorithm-assisted. We evaluated the two approaches based on a structured analysis of different tasks in visual sensitivity analysis as well as the feedback of domain experts. While the work was carried out in the context of epidemiological modeling, the two approaches developed in this work are directly applicable to a variety of modeling processes featuring time series outputs, and can be extended to work with models with other types of outputs.