Self-Supervised Color-Concept Association via Image Colorization
Ruizhen Hu, Ziqi Ye, Bin Chen, Oliver van Kaick, Hui Huang
View presentation:2022-10-19T16:09:00ZGMT-0600Change your timezone on the schedule page
2022-10-19T16:09:00Z
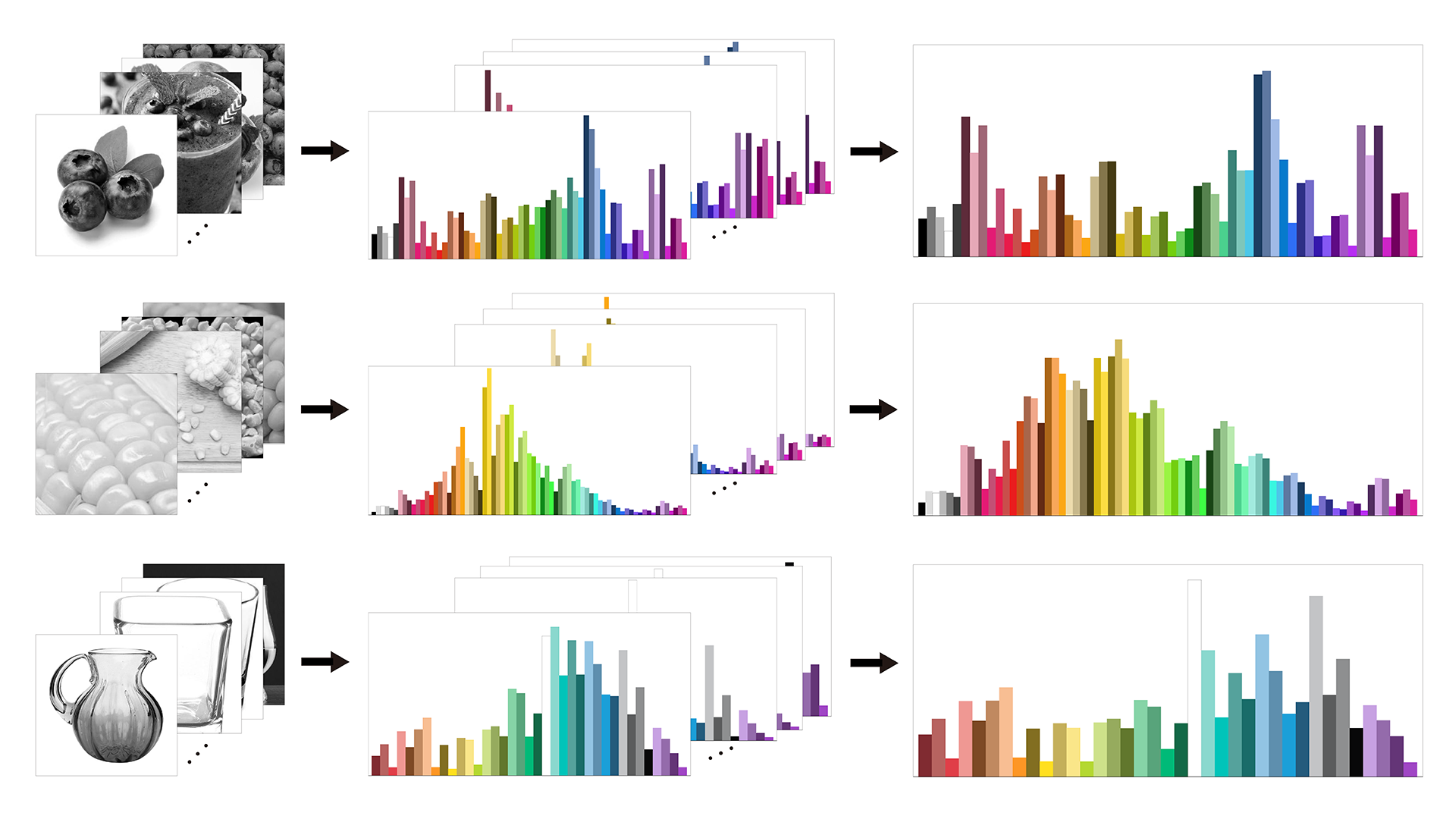
Prerecorded Talk
The live footage of the talk, including the Q&A, can be viewed on the session page, Visualization Opportunities.
Fast forward
Abstract
The interpretation of colors in visualizations is facilitated when the assignments between colors and concepts in the visualizations match human’s expectations, implying that the colors can be interpreted in a semantic manner. However, manually creating a dataset of suitable associations between colors and concepts for use in visualizations is costly, as such associations would have to be collected from humans for a large variety of concepts. To address the challenge of collecting this data, we introduce a method to extract color-concept associations automatically from a set of concept images. While the state-of-the-art method extracts associations from data with supervised learning, we developed a self-supervised method based on colorization that does not require the preparation of ground truth color-concept associations. Our key insight is that a set of images of a concept should be sufficient for learning color-concept associations, since humans also learn to associate colors to concepts mainly from past visual input. Thus, we propose to use an automatic colorization method to extract statistical models of the color-concept associations that appear in concept images. Specifically, we take a colorization model pre-trained on ImageNet and fine-tune it on the set of images associated with a given concept, to predict pixel-wise probability distributions in Lab color space for the images. Then, we convert the predicted probability distributions into color ratings for a given color library and aggregate them for all the images of a concept to obtain the final color-concept associations. We evaluate our method using four different evaluation metrics and via a user study. Experiments show that, although the state-of-the-art method based on supervised learning with user-provided ratings is more effective at capturing relative associations, our self-supervised method obtains overall better results according to metrics like Earth Mover’s Distance (EMD) and Entropy Difference (ED), which are closer to human perception of color distributions.