Interactive Visual Cluster Analysis by Contrastive Dimensionality Reduction
Jiazhi Xia, Linquan Huang, Weixing Lin, Xin Zhao, Jing Wu, Yang Chen, Ying Zhao, Wei Chen
View presentation:2022-10-20T14:36:00ZGMT-0600Change your timezone on the schedule page
2022-10-20T14:36:00Z
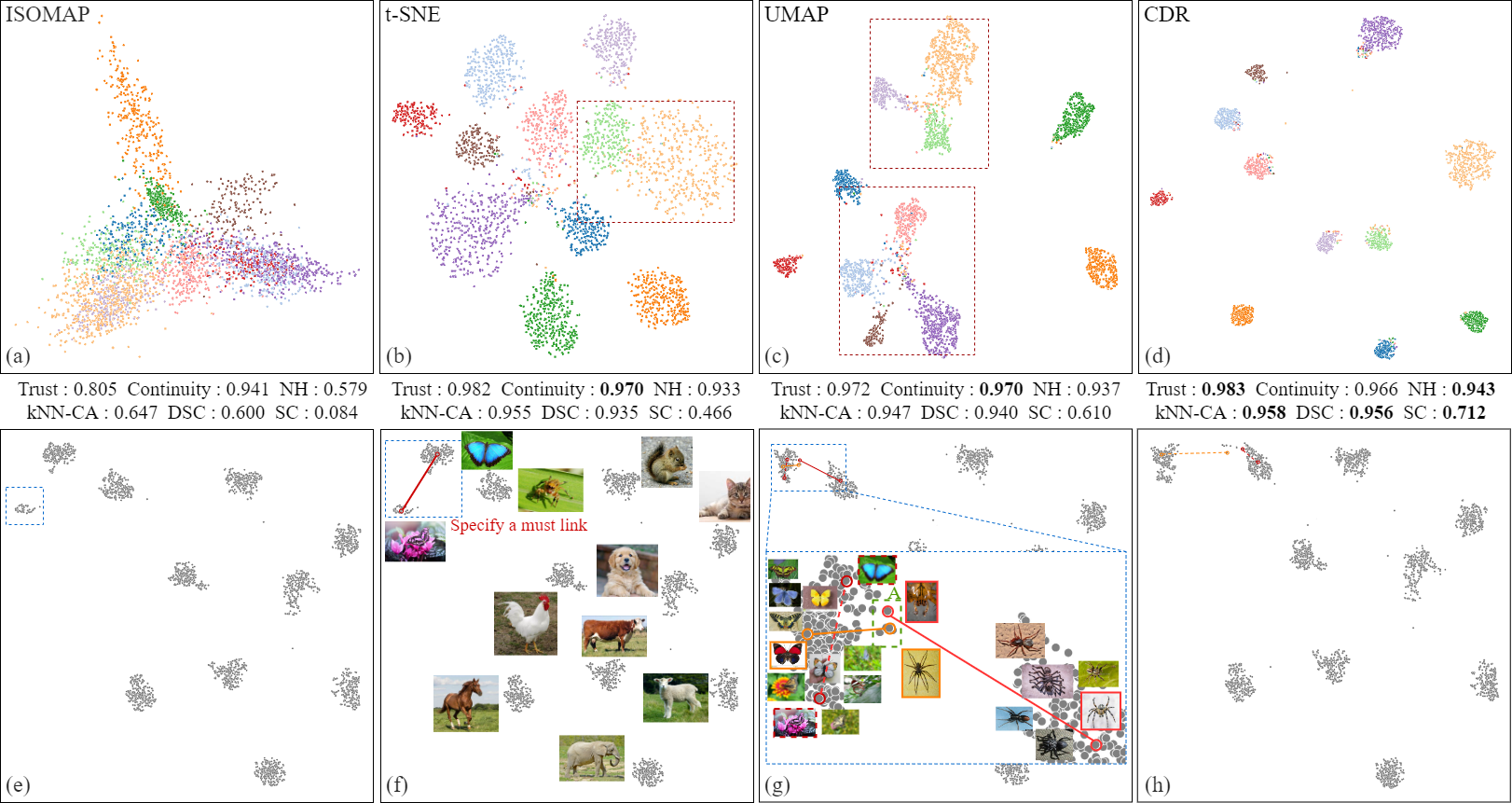
Prerecorded Talk
The live footage of the talk, including the Q&A, can be viewed on the session page, Interactive Dimensionality (High Dimensional Data).
Fast forward
Abstract
We propose a contrastive dimensionality reduction approach (CDR) for interactive visual cluster analysis. Although dimensionality reduction of high-dimensional data is widely used in visual cluster analysis in conjunction with scatterplots, there are several limitations on effective visual cluster analysis. First, it is non-trivial for an embedding to present clear visual cluster separation when keeping neighborhood structures. Second, as cluster analysis is a subjective task, user steering is required. However, it is also non-trivial to enable interactions in dimensionality reduction. To tackle these problems, we introduce contrastive learning into dimensionality reduction for high-quality embedding. We then redefine the gradient of the loss function to the negative pairs to enhance the visual cluster separation of embedding results. Based on the contrastive learning scheme, we employ link-based interactions to steer embeddings. After that, we implement a prototype visual interface that integrates the proposed algorithms and a set of visualizations. Quantitative experiments demonstrate that CDR outperforms existing techniques in terms of preserving correct neighborhood structures and improving visual cluster separation. The ablation experiment demonstrates the effectiveness of gradient redefinition. The user study verifies that CDR outperforms t-SNE and UMAP in the task of cluster identification. We also showcase two use cases on real-world datasets to present the effectiveness of link-based interactions.