Diverse Interaction Recommendation for Public Users Exploring Multi-view Visualization using Deep Learning
Yixuan Li, Yusheng Qi, Yang Shi, Qing Chen, Nan Cao, Siming Chen
View presentation:2022-10-19T14:48:00ZGMT-0600Change your timezone on the schedule page
2022-10-19T14:48:00Z
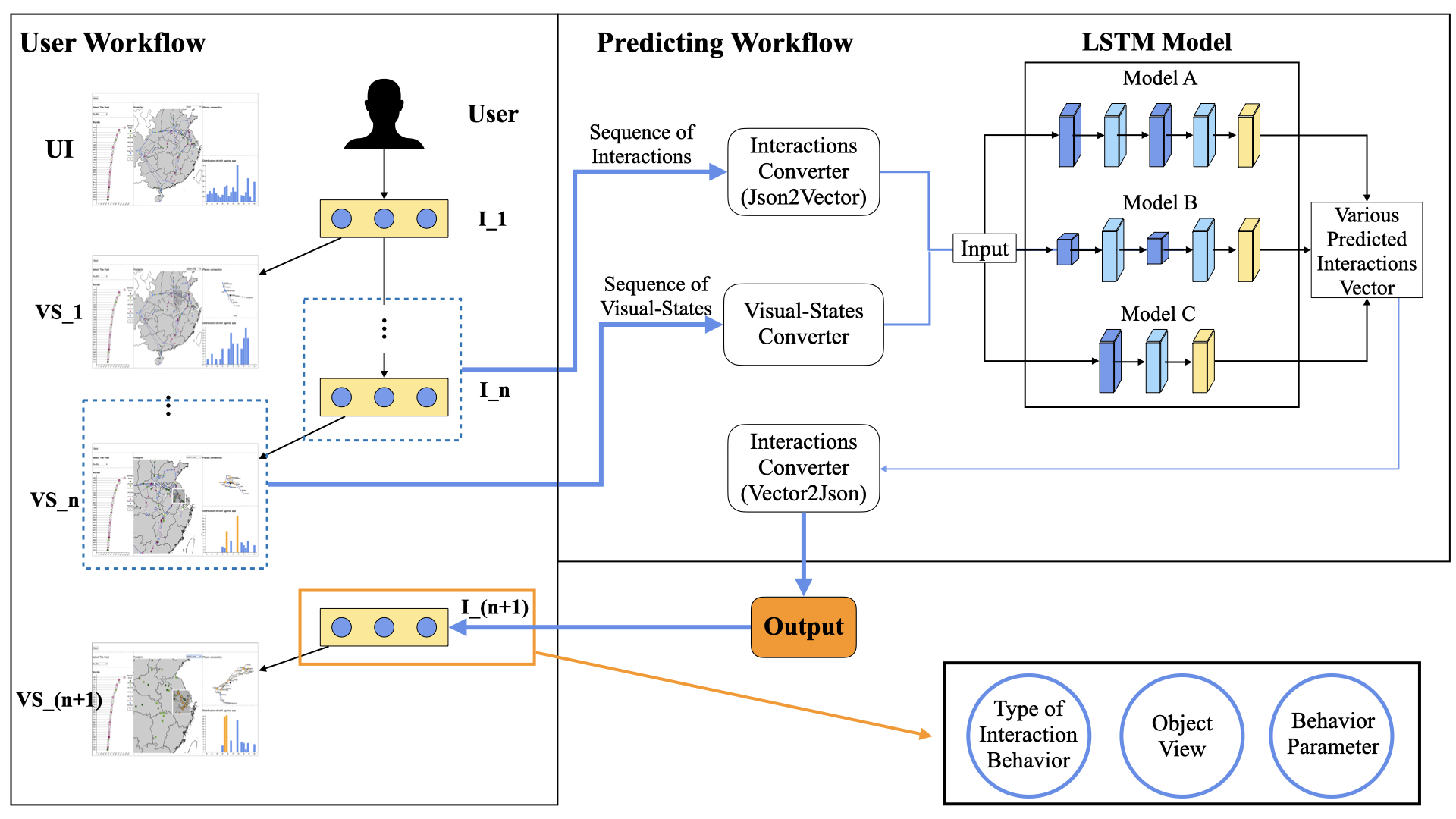
Prerecorded Talk
The live footage of the talk, including the Q&A, can be viewed on the session page, VA and ML.
Fast forward
Abstract
Interaction is an important channel to offer users insights in interactive visualization systems. However, which interaction to operate and which part of data to explore are hard questions for public users facing a multi-view visualization for the first time. Making these decisions largely relies on professional experience and analytic abilities, which is a huge challenge for non-professionals. To solve the problem, we propose a method aiming to provide diverse, insightful, and real-time interaction recommendations for novice users. Building on the Long-Short Term Memory Model (LSTM) structure, our model captures users' interactions and visual states and encodes them in numerical vectors to make further recommendations. Through an illustrative example of a visualization system about Chinese poets in the museum scenario, the model is proven to be workable in systems with multi-views and multiple interaction types. A further user study demonstrates the method's capability to help public users conduct more insightful and diverse interactive explorations and gain more accurate data insights.