RankAxis: Towards a Systematic Combination of Projection and Ranking in Multi-Attribute Data Exploration
Qiangqiang Liu, Yukun Ren, Zhihua Zhu, Dai Li, Xiaojuan Ma, Quan Li
View presentation:2022-10-20T14:00:00ZGMT-0600Change your timezone on the schedule page
2022-10-20T14:00:00Z
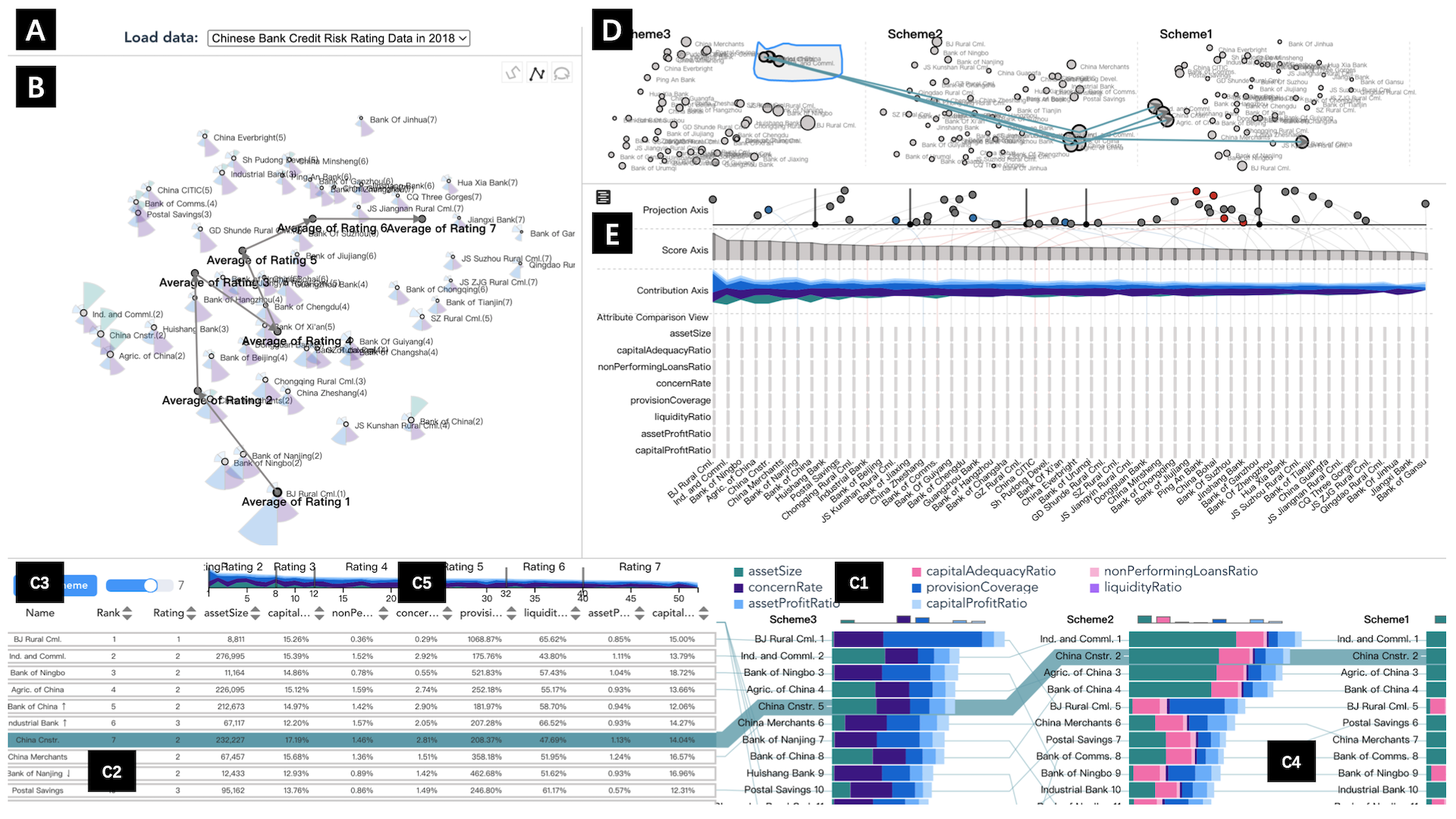
Prerecorded Talk
The live footage of the talk, including the Q&A, can be viewed on the session page, Interactive Dimensionality (High Dimensional Data).
Fast forward
Abstract
Projection and ranking are frequently used analysis techniques in multi-attribute data exploration. Both families of techniques help analysts with tasks such as identifying similarities between observations and determining ordered subgroups, and have shown good performances in multi-attribute data exploration. However, they often exhibit problems such as distorted projection layouts, obscure semantic interpretations, and non-intuitive effects produced by selecting a subset of (weighted) attributes. Moreover, few studies have attempted to combine projection and ranking into the same exploration space to complement each other's strengths and weaknesses. For this reason, we propose RankAxis, a visual analytics system that systematically combines projection and ranking to facilitate the mutual interpretation of these two techniques and jointly support multi-attribute data exploration. A real-world case study, expert feedback, and a user study demonstrate the efficacy of RankAxis.