A Design Space for Surfacing Content Recommendations in Visual Analytic Platforms
Zhilan Zhou, Wenyuan Wang, Mengtian Guo, Yue Wang, David Gotz
View presentation:2022-10-19T14:36:00ZGMT-0600Change your timezone on the schedule page
2022-10-19T14:36:00Z
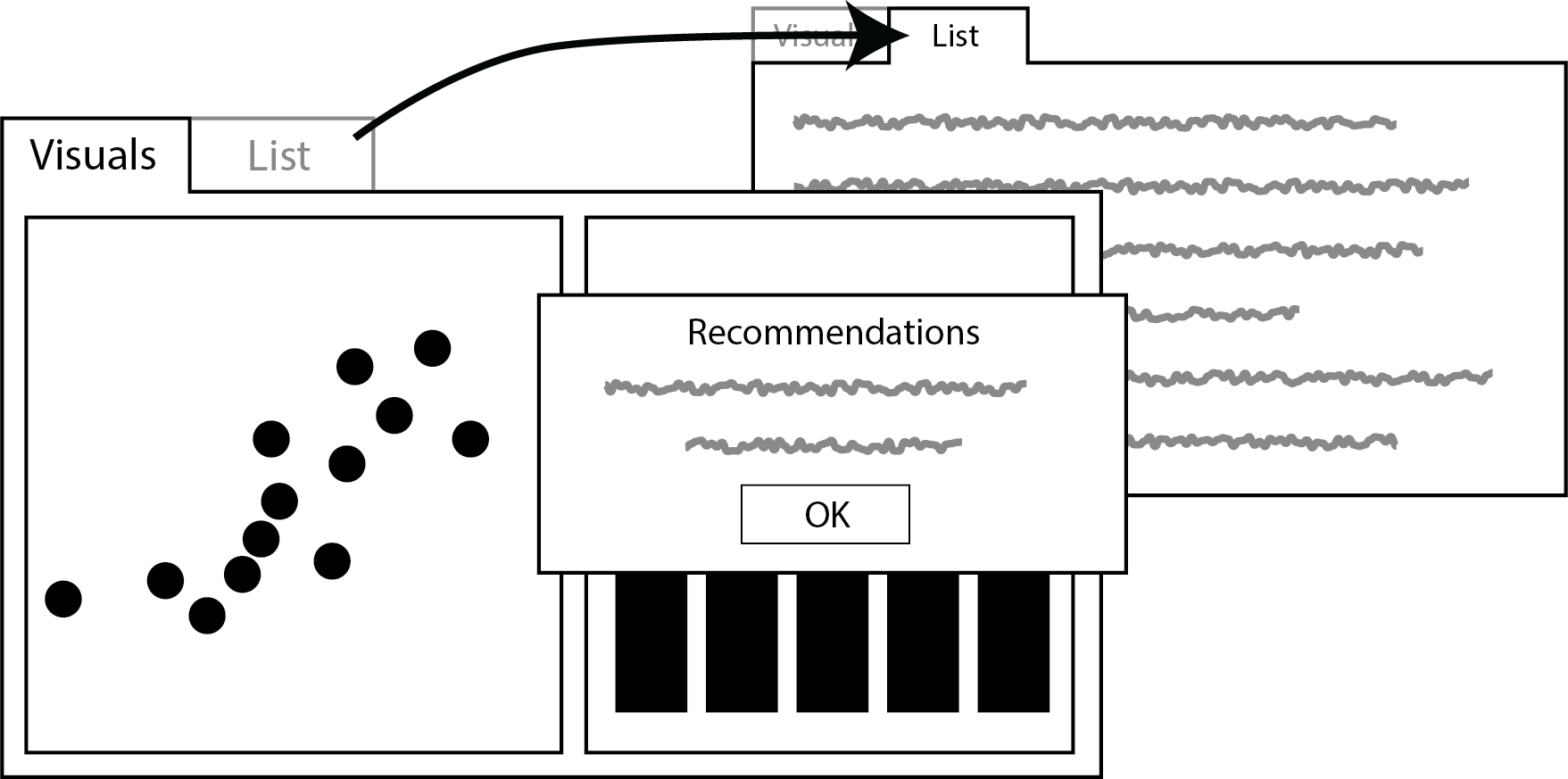
Prerecorded Talk
The live footage of the talk, including the Q&A, can be viewed on the session page, VA and ML.
Fast forward
Abstract
Recommendation algorithms have been leveraged in various ways within visualization systems to assist users as they perform of a range of information tasks. One common focus for these techniques has been the recommendation of content, rather than visual form, as a means to assist users in the identification of information that is relevant to their task context. A wide variety of techniques have been proposed to address this general problem, with a range of design choices in how these solutions surface relevant information to users. This paper reviews the state-of-the-art in how visualization systems surface recommended content to users during users' visual analysis; introduces a four-dimensional design space for visual content recommendation based on a characterization of prior work; and discusses key observations regarding common patterns and future research opportunities.