Interactive and visual prompt engineering for ad-hoc task adaptation with large language models
Hendrik Strobelt, Albert Webson, Victor Sanh, Benjamin Hoover, Johanna Beyer, Hanspeter Pfister, Alexander Rush
View presentation:2022-10-20T21:33:00ZGMT-0600Change your timezone on the schedule page
2022-10-20T21:33:00Z
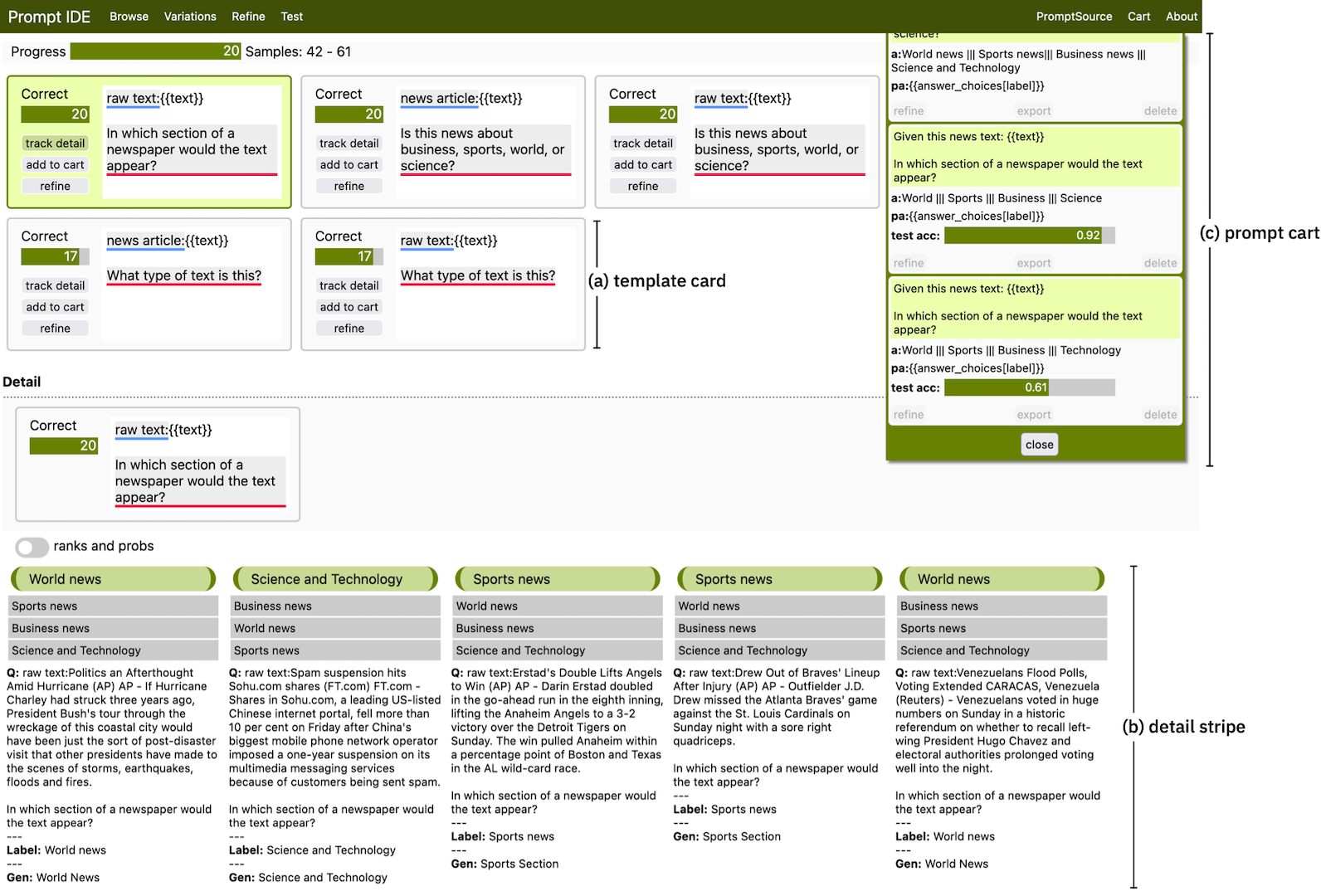
Prerecorded Talk
The live footage of the talk, including the Q&A, can be viewed on the session page, Text, Language, and Image Data.
Fast forward
Abstract
State-of-the-art neural language models can now be used to solve ad-hoc language tasks through zero-shot prompting without the need for supervised training. This approach has gained popularity in recent years, and researchers have demonstrated prompts that achieve strong accuracy on specific NLP tasks. However, finding a prompt for new tasks requires experimentation. Different prompt templates with different wording choices lead to significant accuracy differences. PromptIDE allows users to experiment with prompt variations, visualize prompt performance, and iteratively optimize prompts. We developed a workflow that allows users to first focus on model feedback using small data before moving on to a large data regime that allows empirical grounding of promising prompts using quantitative measures of the task. The tool then allows easy deployment of the newly created ad-hoc models. We demonstrate the utility of PromptIDE (demo: http://prompt.vizhub.ai) and our workflow using several real-world use cases.