Visinity: Visual Spatial Neighborhood Analysis for Multiplexed Tissue Imaging Data
Simon Alexander Warchol, Robert Krüger, Ajit Johnson Nirmal, Giorgio Gaglia, Jared Jessup Jessup, Cecily C. Ritch, John Hoffer, Jeremy Muhlich, Megan L Burger, Tyler Jacks, Sandro Santagata Santagata, Peter Sorger, Hanspeter Pfister
View presentation:2022-10-19T15:00:00ZGMT-0600Change your timezone on the schedule page
2022-10-19T15:00:00Z
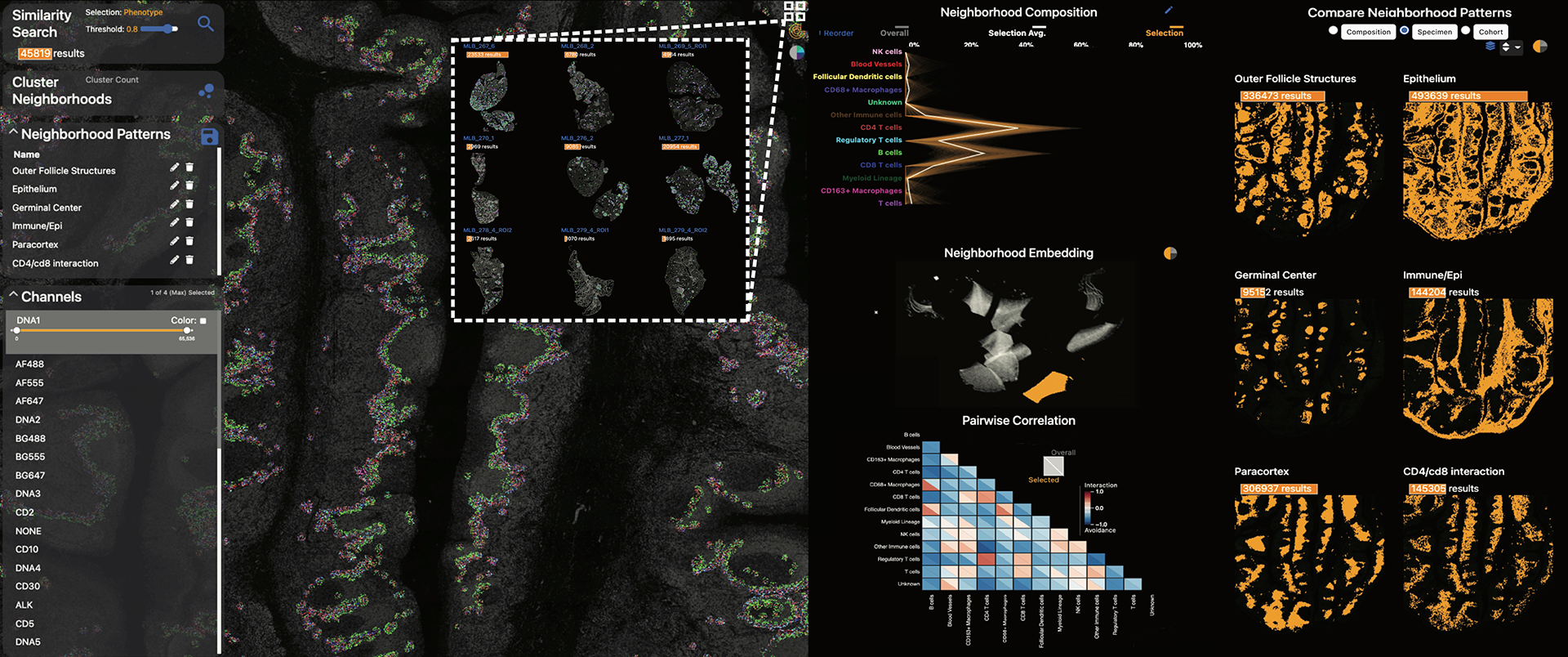
Prerecorded Talk
The live footage of the talk, including the Q&A, can be viewed on the session page, VA and ML.
Fast forward
Abstract
New highly-multiplexed imaging technologies have enabled the study of tissues in unprecedented detail. These methods are increasingly being applied to understand how cancer cells and immune response change during tumor development, progression, and metastasis, as well as following treatment. Yet, existing analysis approaches focus on investigating small tissue samples on a per-cell basis, not taking into account the spatial proximity of cells, which indicates cell-cell interaction and specific biological processes in the larger cancer microenvironment. We present Visinity, a scalable visual analytics system to analyze cell interaction patterns across cohorts of whole-slide multiplexed tissue images. Our approach is based on a fast regional neighborhood computation, leveraging unsupervised learning to quantify, compare, and group cells by their surrounding cellular neighborhood. These neighborhoods can be visually analyzed in an exploratory and confirmatory workflow. Users can explore spatial patterns present across tissues through a scalable image viewer and coordinated views highlighting the neighborhood composition and spatial arrangements of cells. To verify or refine existing hypotheses, users can query for specific patterns to determine their presence and statistical significance. Findings can be interactively annotated, ranked, and compared in the form of small multiples. In two case studies with biomedical experts, we demonstrate that Visinity can identify common biological processes within a human tonsil and uncover novel white-blood cell networks and immune-tumor interactions.