Uniform Manifold Approximation with Two-phase Optimization
Hyeon Jeon, Hyung-Kwon Ko, Soohyun Lee, Jaemin Jo, Jinwook Seo
View presentation:2022-10-19T21:48:00ZGMT-0600Change your timezone on the schedule page
2022-10-19T21:48:00Z
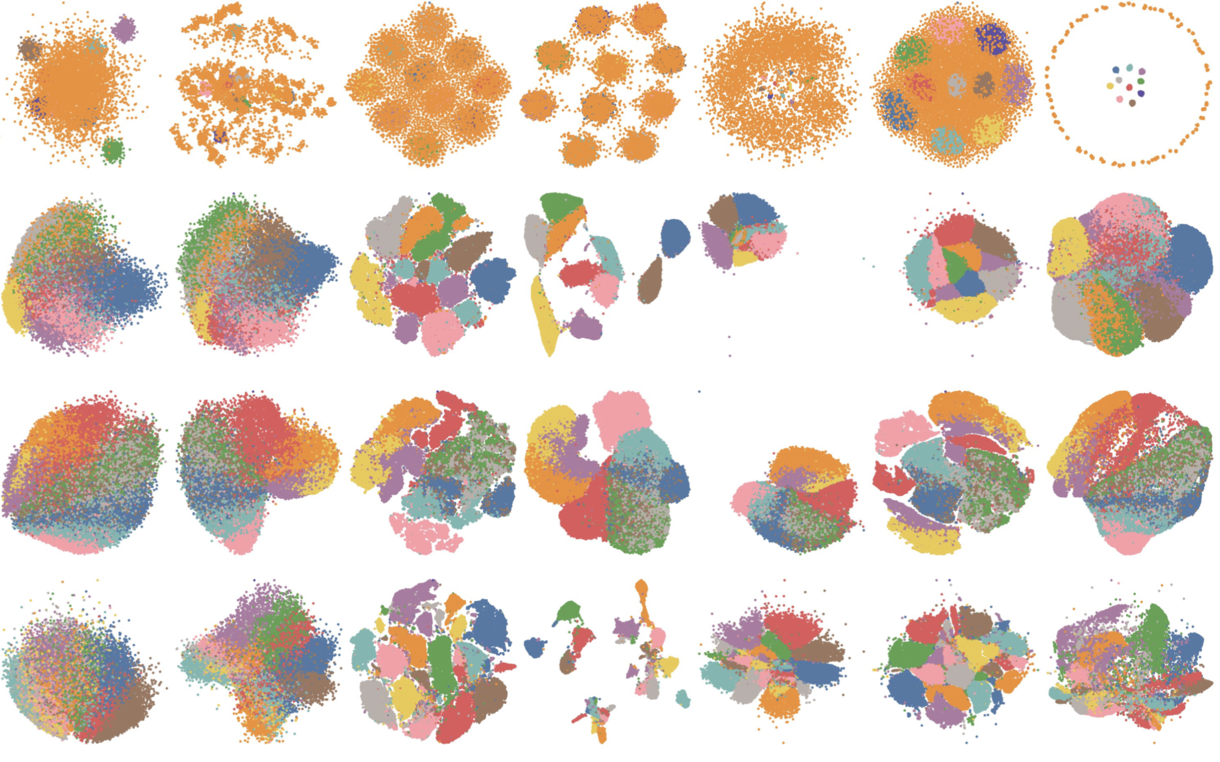
Prerecorded Talk
The live footage of the talk, including the Q&A, can be viewed on the session page, Visual Analytics, Decision Support, and Machine Learning.
Fast forward
Keywords
Human-centered computing—Visualization—Visualization techniques; Computing methodologies—Machine learning—Machine learning algorithms
Abstract
We introduce Uniform Manifold Approximation with Two-phase Optimization (UMATO), a dimensionality reduction (DR) technique that improves UMAP to capture the global structure of high-dimensional data more accurately. In UMATO, optimization is divided into two phases so that the resulting embeddings can depict the global structure reliably while preserving the local structure with sufficient accuracy. In the first phase, hub points are identified and projected to construct a skeletal layout for the global structure. In the second phase, the remaining points are added to the embedding preserving the regional characteristics of local areas. Through quantitative experiments, we found that UMATO (1) outperformed widely used DR techniques in preserving the global structure while (2) producing competitive accuracy in representing the local structure. We also verified that UMATO is preferable in terms of robustness over diverse initialization methods, numbers of epochs, and subsampling techniques.