Volume Puzzle: visual analysis of segmented volume data with multivariate attributes
Marco Agus, Amal Aboulhassan, Khaled Ahmed Lutf Al-Thelaya, Giovanni Pintore, Enrico Gobbetti, Corrado Cali', Jens Schneider
View presentation:2022-10-20T19:09:00ZGMT-0600Change your timezone on the schedule page
2022-10-20T19:09:00Z
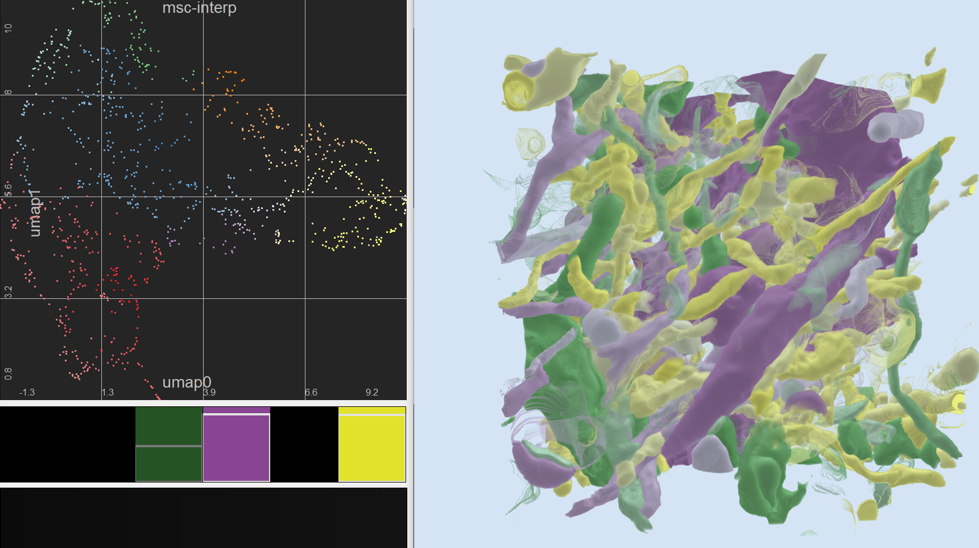
Prerecorded Talk
The live footage of the talk, including the Q&A, can be viewed on the session page, Scientific Visualization, Ensembles, and Accessibility.
Fast forward
Keywords
Segmented Volumes, Multivariate data, Color mapping, Dimensionality reduction
Abstract
A variety of application domains, including material science, neuroscience, and connectomics, commonly use segmented volume data for explorative visual analysis. In many cases, segmented objects are characterized by multivariate attributes expressing specific geometric or physical features. Objects with similar characteristics, determined by selected attribute configurations, can create peculiar spatial patterns, whose detection and study is of fundamental importance. This task is notoriously difficult, especially when the number of attributes per segment is large. In this work, we propose an interactive framework that combines a state-of-the-art direct volume renderer for categorical volumes with techniques for the analysis of the attribute space and for the automatic creation of 2D transfer function. We show, in particular, how dimensionality reduction, kernel-density estimation, and topological techniques such as Morse analysis combined with scatter and density plots allow the efficient design of two-dimensional color maps that highlight spatial patterns. The capabilities of our framework are demonstrated on synthetic and real-world data from several domains.