Visualizing Rule-based Classifiers for Clinical Risk Prognosis
Dario Antweiler, Georg Fuchs
View presentation:2022-10-19T21:03:00ZGMT-0600Change your timezone on the schedule page
2022-10-19T21:03:00Z
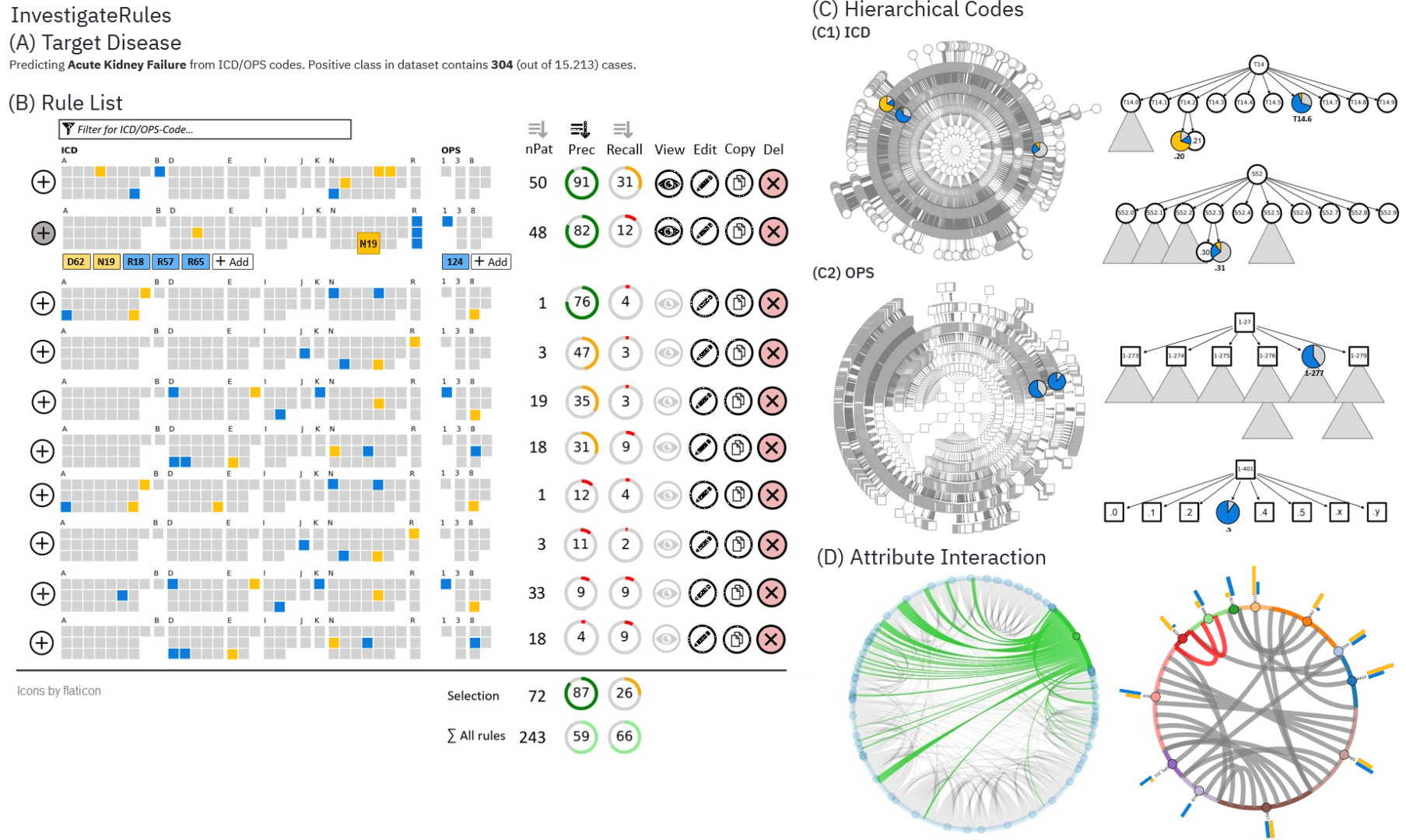
Prerecorded Talk
The live footage of the talk, including the Q&A, can be viewed on the session page, Visual Analytics, Decision Support, and Machine Learning.
Fast forward
Keywords
Information systems applications, Decision support systems, Data analytics, Human computer interaction (HCI), HCI design and evaluation methods, User studies, Applied computing, Life and medical sciences, Health care information systems
Abstract
Deteriorating conditions in hospital patients are a major factor in clinical patient mortality. Currently, timely detection is based on clinical experience, expertise, and attention. However, healthcare trends towards larger patient cohorts, more data, and the desire for better and more personalized care are pushing the existing, simple scoring systems to their limits. Data-driven approaches can extract decision rules from available medical coding data, which offer good interpretability and thus are key for successful adoption in practice. Before deployment, models need to be scrutinized by domain experts to identify errors and check them against existing medical knowledge. We propose a visual analytics system to support healthcare professionals in inspecting and enhancing rule-based classifier through identification of similarities and contradictions, as well as modification of rules. This work was developed iteratively in close collaboration with medical professionals. We discuss how our tool supports the inspection and assessment of rule-based classifiers in the clinical coding domain and propose possible extensions.