RCMVis: A Visual Analytics System for Route Choice Modeling
DongHwa Shin, Jaemin Jo, Bohyoung Kim, Hyunjoo Song, Shin-Hyung Cho, Jinwook Seo
View presentation:2022-10-20T21:45:00ZGMT-0600Change your timezone on the schedule page
2022-10-20T21:45:00Z
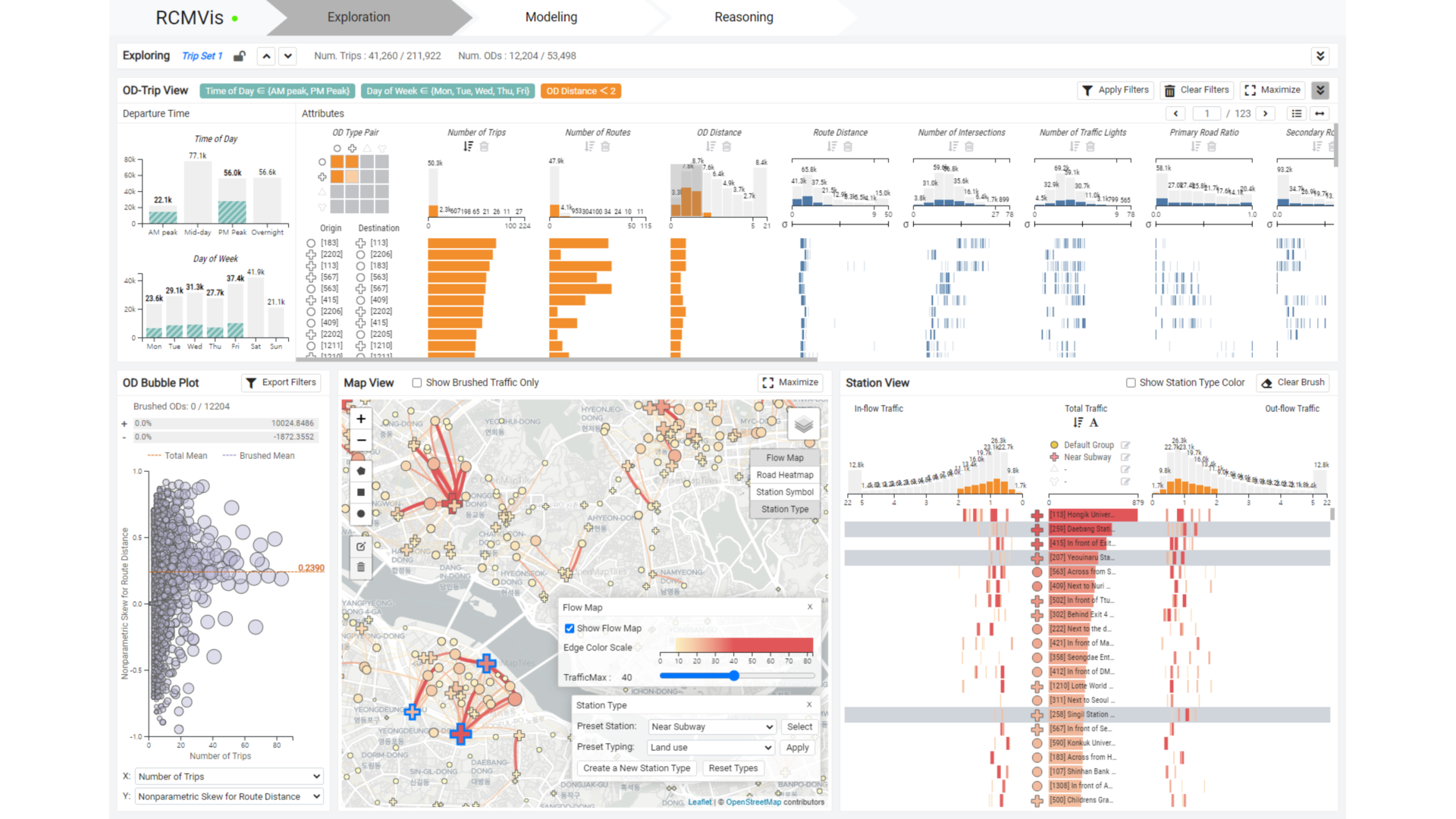
Prerecorded Talk
The live footage of the talk, including the Q&A, can be viewed on the session page, Infrastructure Management.
Fast forward
Keywords
route choice modeling, urban planning, trajectory data, origin-destination, visual analytics
Abstract
We present RCMVis, a visual analytics system to support interactive Route Choice Modeling analysis. It aims to model which characteristics of routes, such as distance and the number of traffic lights, affect travelers' route choice behaviors and how much they affect the choice during their trips. Through close collaboration with domain experts, we designed a visual analytics framework for Route Choice Modeling. The framework supports three interactive analysis stages: exploration, modeling, and reasoning. In the exploration stage, we help analysts interactively explore trip data from multiple origin-destination (OD) pairs and choose a subset of data they want to focus on. To this end, we provide coordinated multiple OD views with different foci that allow analysts to inspect, rank, and compare OD pairs in terms of their multidimensional attributes. In the modeling stage, we integrate a k-medoids clustering method and a path-size logit model into our system to enable analysts to model route choice behaviors from trips with support for feature selection, hyperparameter tuning, and model comparison. Finally, in the reasoning stage, we help analysts rationalize and refine the model by selectively inspecting the trips that strongly support the modeling result. For evaluation, we conducted a case study and interviews with domain experts. The domain experts discovered unexpected insights from numerous modeling results, allowing them to explore the hyperparameter space more effectively to gain better results. In addition, they gained OD- and road-level insights into which data mainly supported the modeling result, enabling further discussion of the model.