Towards Better Caption Supervision for Object Detection
Changjian Chen, Jing Wu, Xiaohan Wang, Shouxing Xiang, Song-Hai Zhang, Qifeng Tang, Shixia Liu
View presentation:2022-10-19T14:12:00ZGMT-0600Change your timezone on the schedule page
2022-10-19T14:12:00Z
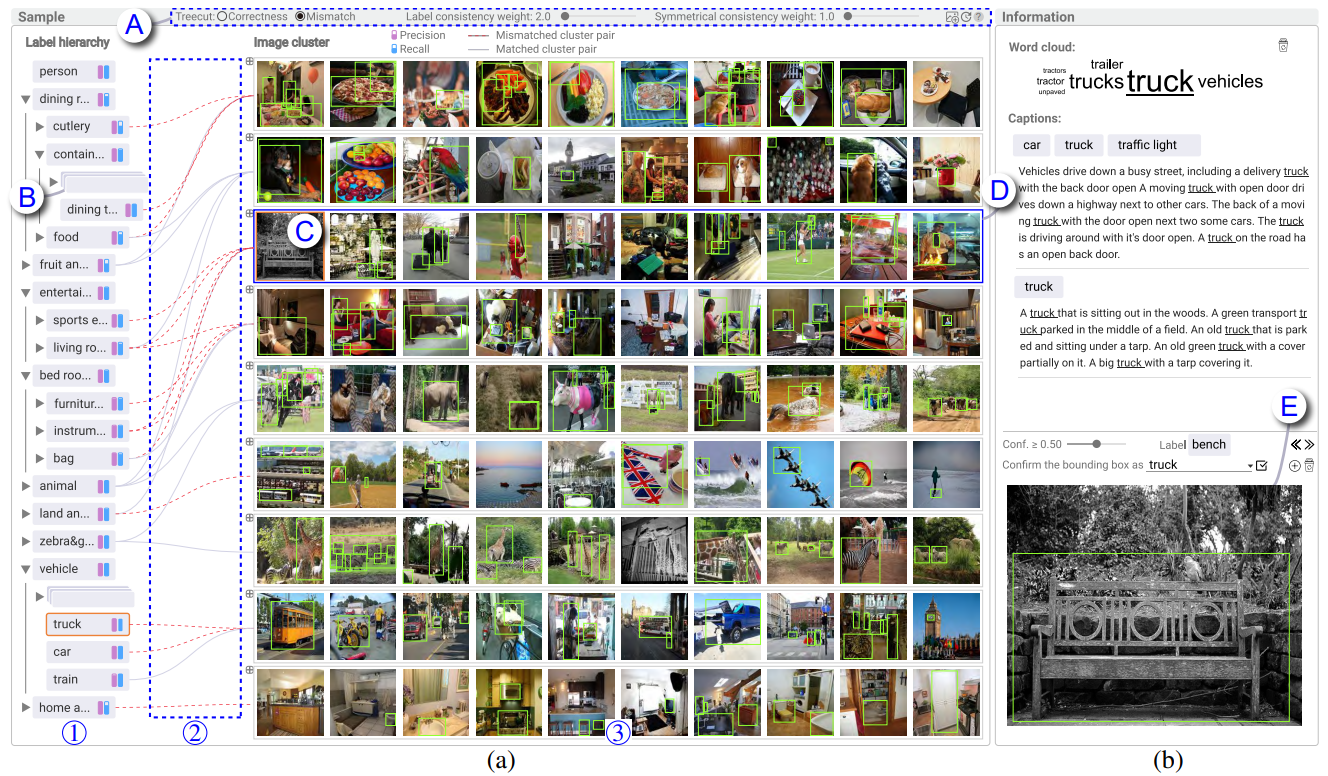
Prerecorded Talk
The live footage of the talk, including the Q&A, can be viewed on the session page, VA and ML.
Fast forward
Keywords
Machine learning, interactive visualization, object detection, caption supervision, co-clustering.
Abstract
As training high-performance object detectors requires expensive bounding box annotations, recent methods resort to free available image captions. However, detectors trained on caption supervision perform poorly because captions are usually noisy and cannot provide precise location information. To tackle this issue, we present a visual analysis method, which tightly integrates caption supervision with object detection to mutually enhance each other. In particular, object labels are first extracted from captions, which are utilized to train the detectors. Then, the label information from images is fed into caption supervision for further improvement. To effectively loop users into the object detection process, a node-link-based set visualization supported by a multi-type relational co-clustering algorithm is developed to explain the relationships between the extracted labels and the images with detected objects. The co-clustering algorithm clusters labels and images simultaneously by utilizing both their representations and their relationships. Quantitative evaluations and a case study are conducted to demonstrate the efficiency and effectiveness of the developed method in improving the performance of object detectors.