Visualizing Graph Neural Networks with CorGIE: Corresponding a Graph to Its Embedding
Zipeng Liu, Yang Wang, Jürgen Bernard, Tamara Munzner
View presentation:2022-10-20T16:09:00ZGMT-0600Change your timezone on the schedule page
2022-10-20T16:09:00Z
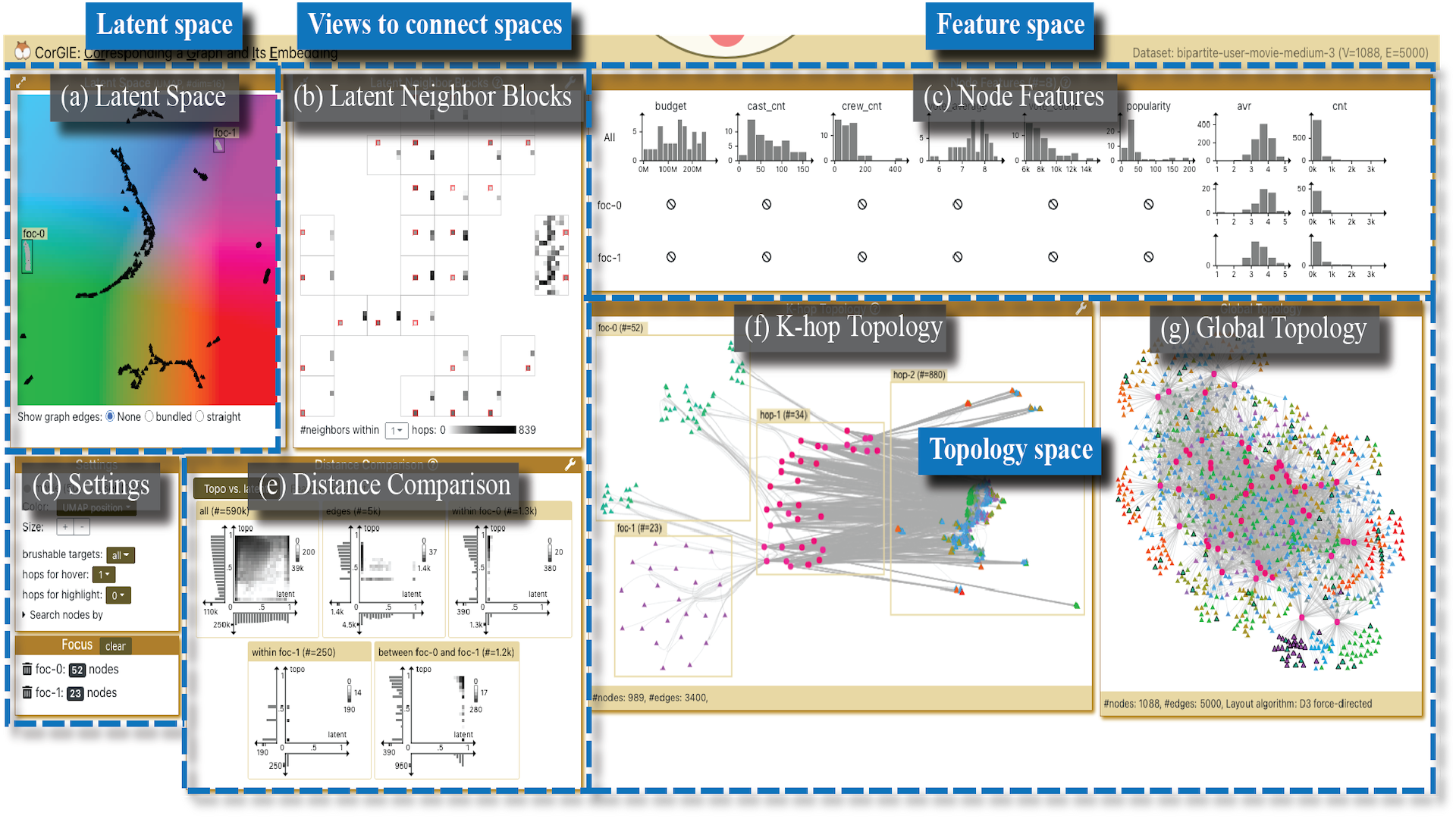
Prerecorded Talk
The live footage of the talk, including the Q&A, can be viewed on the session page, Graphs and Networks.
Fast forward
Keywords
Visualization for machine learning, graph neural network, graph layout
Abstract
Graph neural networks (GNNs) are a class of powerful machine learning tools that model node relations for making predictions of nodes or links. GNN developers rely on quantitative metrics of the predictions to evaluate a GNN, but similar to many other neural networks, it is difficult for them to understand if the GNN truly learns characteristics of a graph as expected. We propose an approach to corresponding an input graph to its node embedding (aka latent space), a common component of GNNs that is later used for prediction. We abstract the data and tasks, and develop an interactive multi-view interface called CorGIE to instantiate the abstraction. As the key function in CorGIE, we propose the K-hop graph layout to show topological neighbors in hops and their clustering structure. To evaluate the functionality and usability of CorGIE, we present how to use CorGIE in two usage scenarios, and conduct a case study with five GNN experts.