Local Latent Representation based on Geometric Convolution for Particle Data Feature Exploration
Haoyu Li, Han-Wei Shen
View presentation:2022-10-19T21:21:00ZGMT-0600Change your timezone on the schedule page
2022-10-19T21:21:00Z
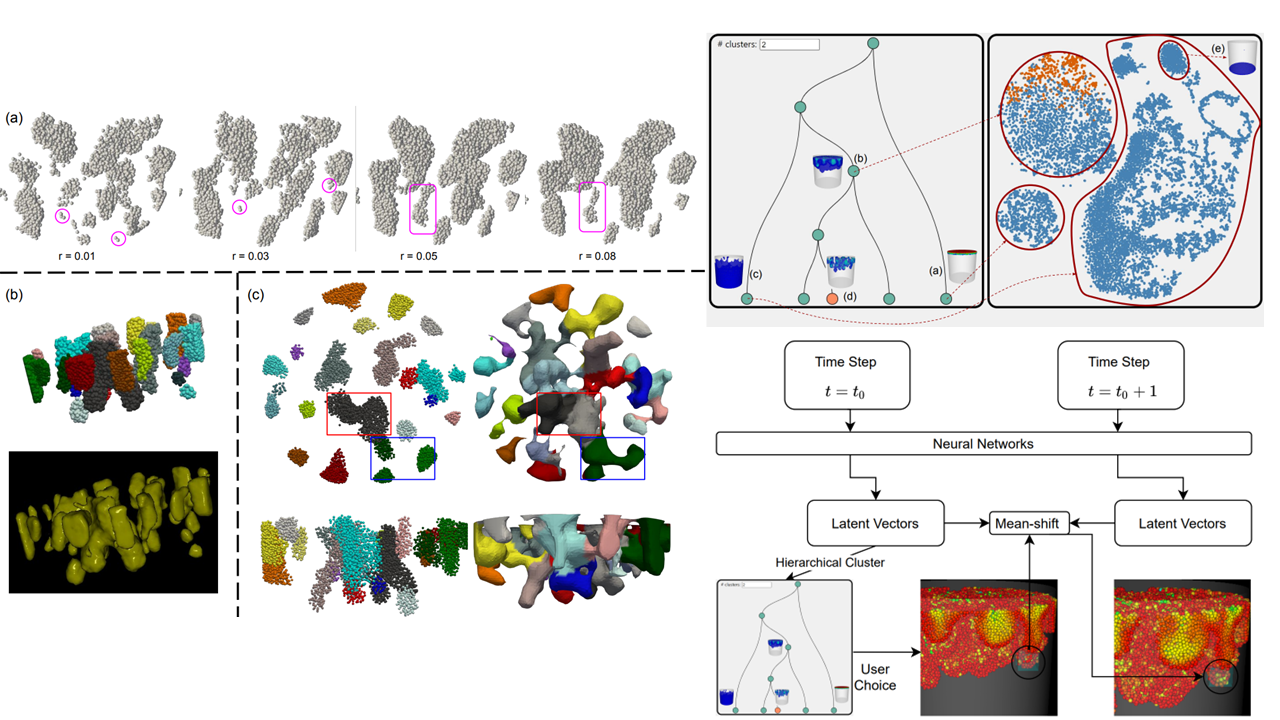
Prerecorded Talk
The live footage of the talk, including the Q&A, can be viewed on the session page, Dealing with Scale, Space, and Dimension.
Fast forward
Keywords
Data transformation, Particle data, Feature extraction and tracking, Deep learning
Abstract
Feature related particle data analysis plays an important role in many scientific applications such as fluid simulations, cosmology simulations and molecular dynamics. Compared to conventional methods that use hand-crafted feature descriptors, some recent studies focus on transforming the data into a new latent space, where features are easier to be identified, compared and extracted. However, it is challenging to transform particle data into latent representations, since the convolution neural networks used in prior studies require the data presented in regular grids. In this paper, we adopt Geometric Convolution, a neural network building block designed for 3D point clouds, to create latent representations for scientific particle data. These latent representations capture both the particle positions and their physical attributes in the local neighborhood so that features can be extracted by clustering in the latent space, and tracked by applying tracking algorithms such as mean-shift. We validate the extracted features and tracking results from our approach using datasets from three applications and show that they are comparable to the methods that define hand-crafted features for each specific dataset.