DL4SciVis: A State-of-the-Art Survey on Deep Learning for Scientific Visualization
Chaoli Wang, Jun Han
View presentation:2022-10-20T14:12:00ZGMT-0600Change your timezone on the schedule page
2022-10-20T14:12:00Z
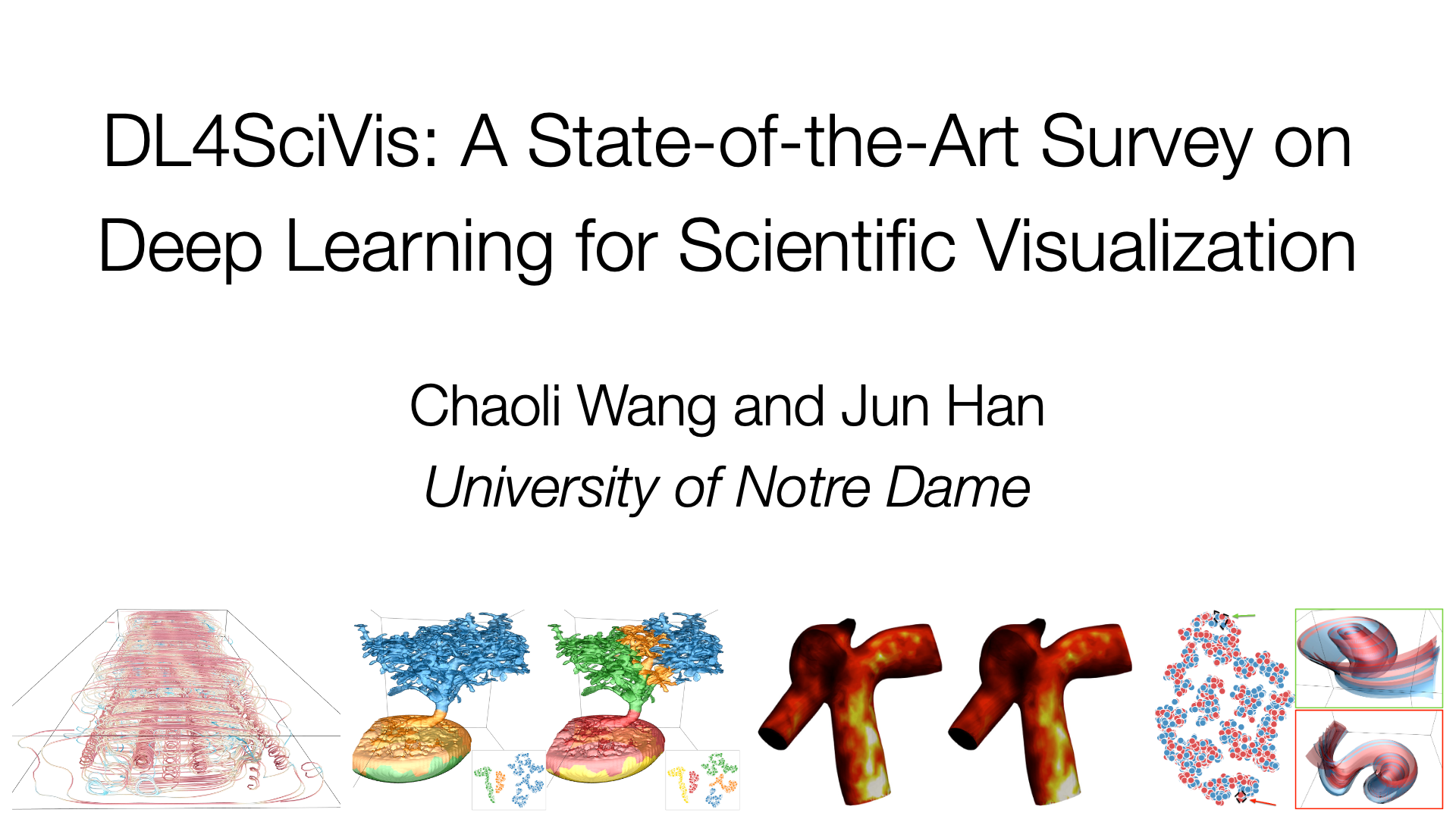
Prerecorded Talk
The live footage of the talk, including the Q&A, can be viewed on the session page, ML for VIS.
Fast forward
Keywords
Scientific visualization, deep learning, survey
Abstract
Since 2016, we have witnessed the tremendous growth of artificial intelligence+visualization (AI+VIS) research. However, existing survey papers on AI+VIS focus on visual analytics and information visualization, not scientific visualization (SciVis). In this paper, we survey related deep learning (DL) works in SciVis, specifically in the direction of DL4SciVis: designing DL solutions for solving SciVis problems. To stay focused, we primarily consider works that handle scalar and vector field data but exclude mesh data. We classify and discuss these works along six dimensions: domain setting, research task, learning type, network architecture, loss function, and evaluation metric. The paper concludes with a discussion of the remaining gaps to fill along the discussed dimensions and the grand challenges we need to tackle as a community. This state-of-the-art survey guides SciVis researchers in gaining an overview of this emerging topic and points out future directions to grow this research.