Diagnosing Ensemble Few-Shot Classifiers
Weikai Yang, Xi Ye, Xingxing Zhang, Lanxi Xiao, Jiazhi Xia, Zhongyuan Wang, Jun Zhu, Hanspeter Pfister, Shixia Liu
View presentation:2022-10-19T16:45:00ZGMT-0600Change your timezone on the schedule page
2022-10-19T16:45:00Z
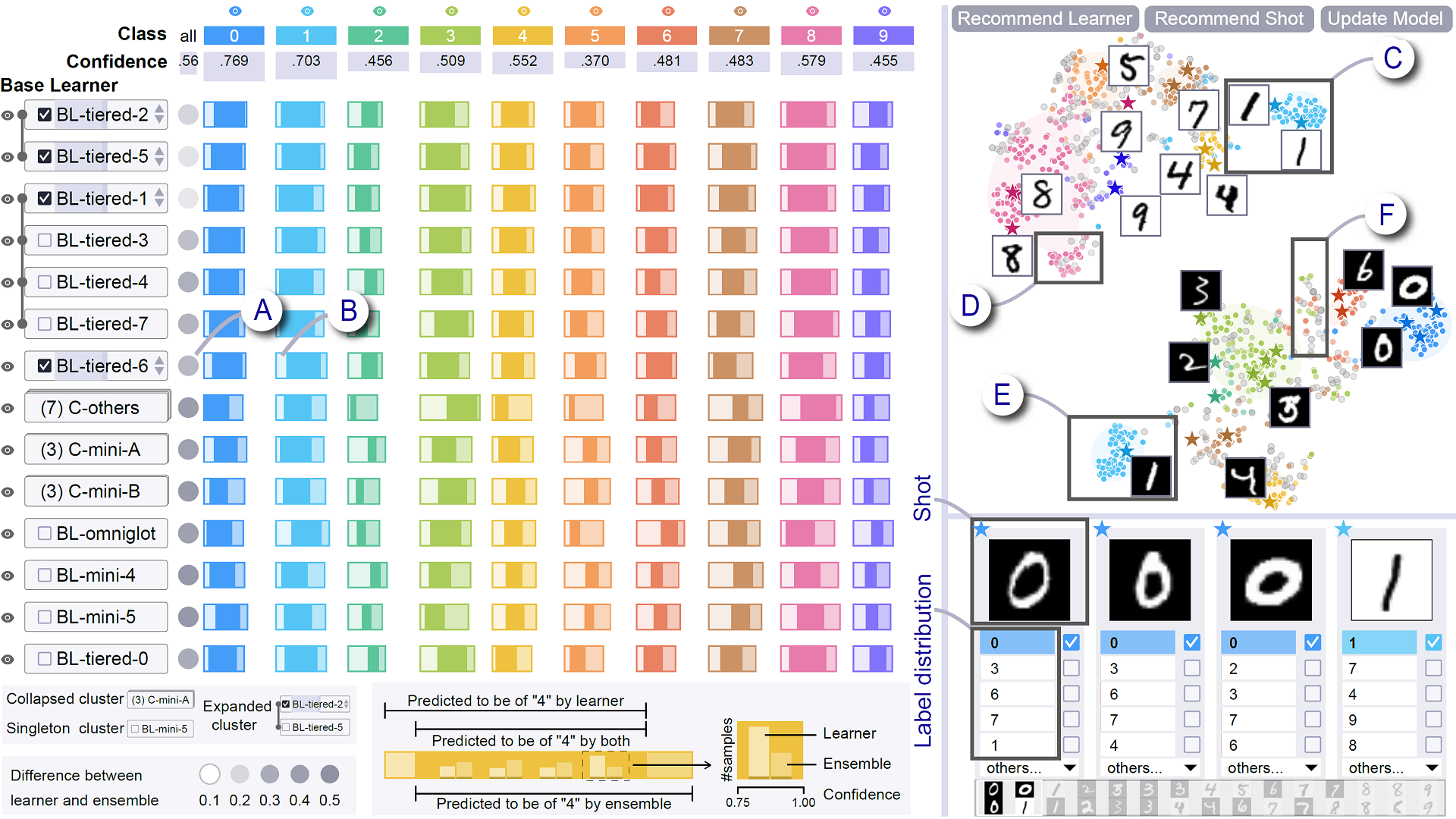
Prerecorded Talk
The live footage of the talk, including the Q&A, can be viewed on the session page, VA for ML.
Fast forward
Keywords
Few-shot learning, ensemble model, subset selection, matrix visualization, scatterplot
Abstract
The base learners and labeled samples (shots) in an ensemble few-shot classifier greatly affect the model performance. When the performance is not satisfactory, it is usually difficult to understand the underlying causes and make improvements. To tackle this issue, we propose a visual analysis method, FSLDiagnotor. Given a set of base learners and a collection of samples with a few shots, we consider two problems: 1) finding a subset of base learners that well predict the sample collections; and 2) replacing the low-quality shots with more representative ones to adequately represent the sample collections. We formulate both problems as sparse subset selection and develop two selection algorithms to recommend appropriate learners and shots, respectively. A matrix visualization and a scatterplot are combined to explain the recommended learners and shots in context and facilitate users in adjusting them. Based on the adjustment, the algorithm updates the recommendation results for another round of improvement. Two case studies are conducted to demonstrate that FSLDiagnotor helps build a few-shot classifier efficiently and increases the accuracy by 12% and 21%, respectively.