EBBE-Text: Explaining Neural Networks by Exploring Text Classification Decision Boundaries
Alexis Delaforge, Jérôme Azé, Sandra Bringay, Caroline Mollevi, Arnaud Sallaberry, Maximilien Servajean
View presentation:2022-10-20T20:57:00ZGMT-0600Change your timezone on the schedule page
2022-10-20T20:57:00Z
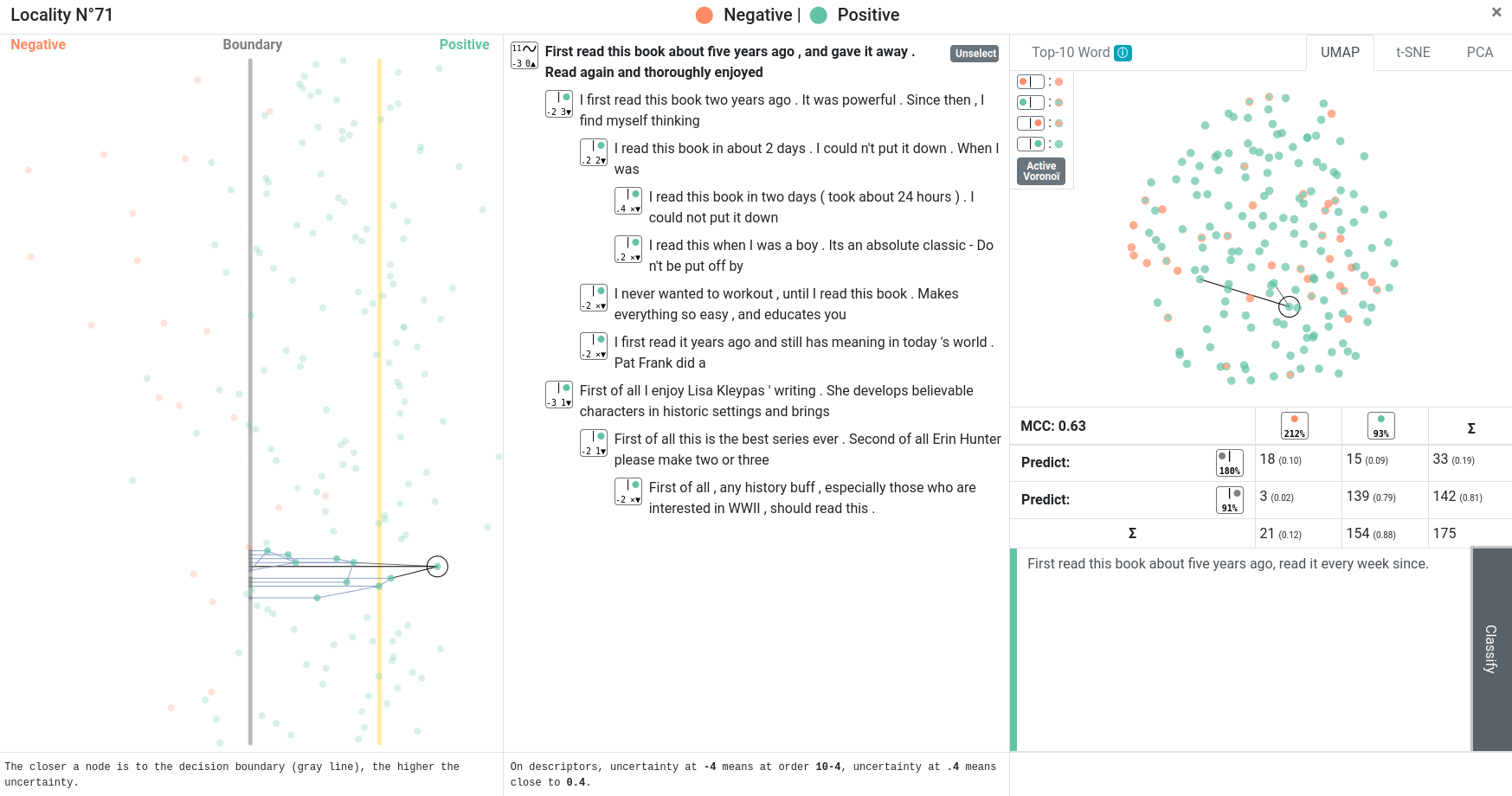
Prerecorded Talk
The live footage of the talk, including the Q&A, can be viewed on the session page, Text, Language, and Image Data.
Fast forward
Keywords
Artificial neural networks, Data visualization, Computational modeling, Natural language processing, Predictive models, Task analysis, Deep learning, Binary text classification, decision boundary, deep learning, interpretability, neural networks, representation space, visual analytics
Abstract
While neural networks (NN) have been successfully applied to many NLP tasks, the way they function is often difficult to interpret. In this article, we focus on binary text classification via NNs and propose a new tool, which includes a visualization of the decision boundary and the distances of data elements to this boundary. This tool increases the interpretability of NN. Our approach uses two innovative views: (1) an overview of the text representation space and (2) a local view allowing data exploration around the decision boundary for various localities of this representation space. These views are integrated into a visual platform, EBBE-Text, which also contains state-of-the-art visualizations of NN representation spaces and several kinds of information obtained from the classification process. The various views are linked through numerous interactive functionalities that enable easy exploration of texts and classification results via the various complementary views. A user study shows the effectiveness of the visual encoding and a case study illustrates the benefits of using our tool for the analysis of the classifications obtained with several recent NNs and two datasets.