A Unified Understanding of Deep NLP Models for Text Classification
Zhen Li, Xiting Wang, Weikai Yang, Jing Wu, Zhengyan Zhang, Zhiyuan Liu, Maosong Sun, Hui Zhang, Shixia Liu
View presentation:2022-10-20T20:45:00ZGMT-0600Change your timezone on the schedule page
2022-10-20T20:45:00Z
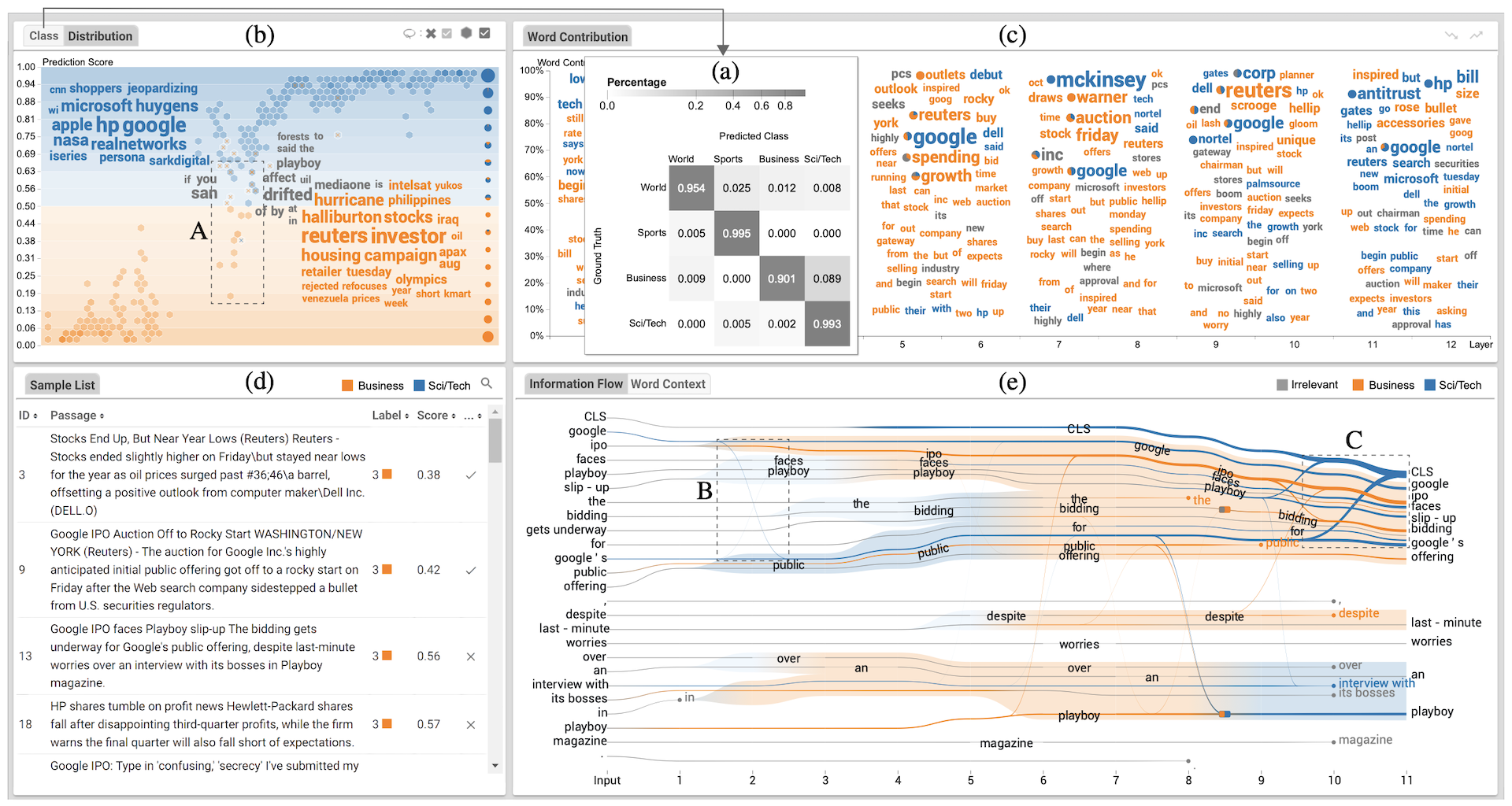
Prerecorded Talk
The live footage of the talk, including the Q&A, can be viewed on the session page, Text, Language, and Image Data.
Fast forward
Keywords
Explainable AI, visual debugging, visual analytics, deep NLP model, information-based interpretation
Abstract
The rapid development of deep natural language processing (NLP) models for text classification has led to an urgent need for a unified understanding of these models proposed individually. Existing methods cannot meet the need for understanding different models in one framework due to the lack of a unified measure for explaining both low-level (e.g., words) and high-level (e.g., phrases) features. We have developed a visual analysis tool, DeepNLPVis, to enable a unified understanding of NLP models for text classification. The key idea is a mutual information-based measure, which provides quantitative explanations on how each layer of a model maintains the information of input words in a sample. We model the intra- and inter-word information at each layer measuring the importance of a word to the final prediction as well as the relationships between words, such as the formation of phrases. A multi-level visualization, which consists of a corpus-level, a sample-level, and a word-level visualization, supports the analysis from the overall training set to individual samples. Two case studies on classification tasks and comparison between models demonstrate that DeepNLPVis can help users effectively identify potential problems caused by samples and model architectures and then make informed improvements.