Subject-Specific Brain Activity Analysis in fMRI Data Using Merge Trees
Farhan Rasheed, Daniel Jönsson, Emma Nilsson, Talha Bin Masood, Ingrid Hotz
View presentation:2022-10-17T21:48:00ZGMT-0600Change your timezone on the schedule page
2022-10-17T21:48:00Z
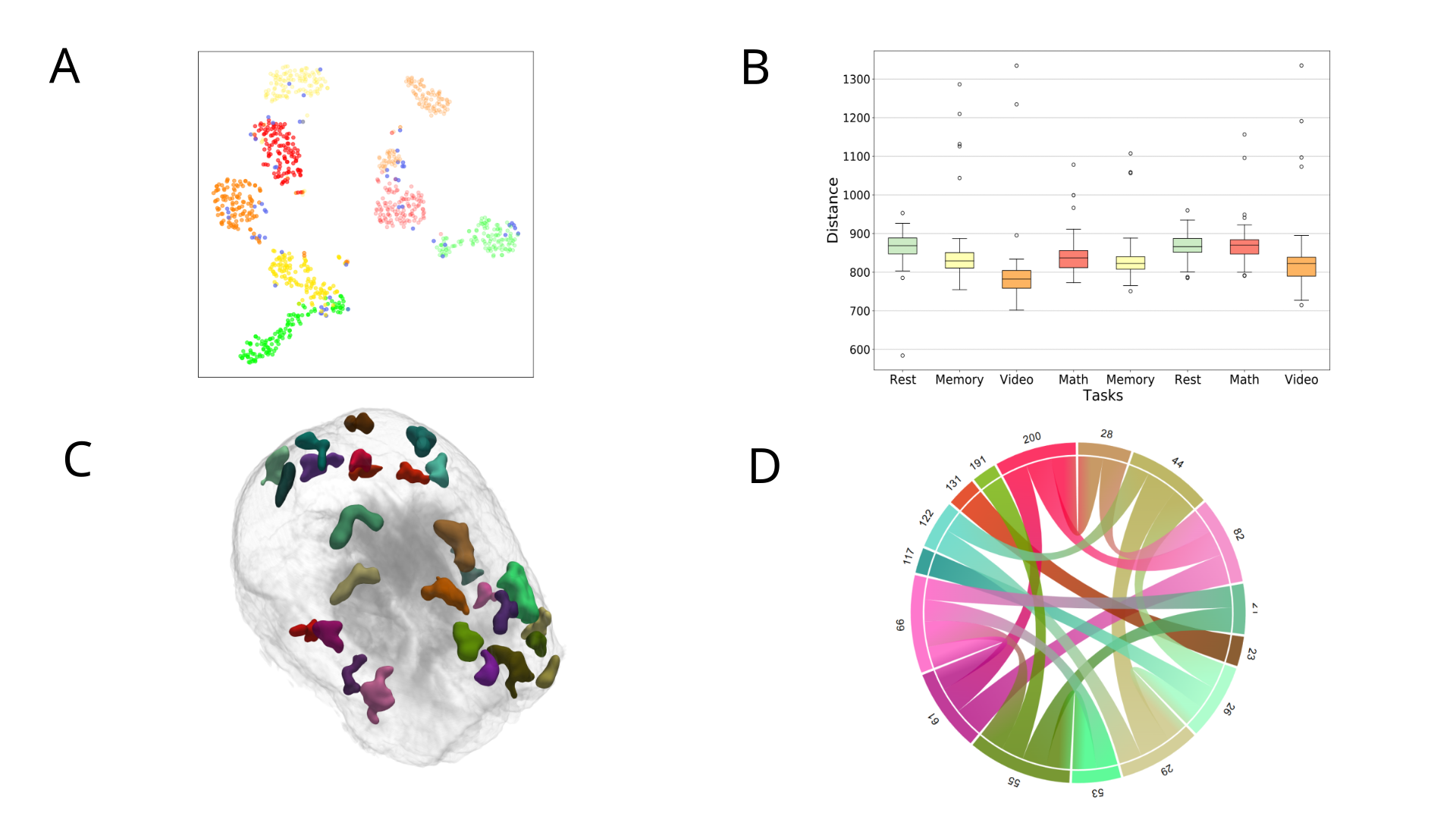
The live footage of the talk, including the Q&A, can be viewed on the session page, TopoInVis: Session 2, Early Career Lightning Talks + Best Paper Awards .
Keywords
fMRI data analysis, data abstraction, temporal data, feature detection, merge tree, computational topology-based techniques
Abstract
We present a method for detecting patterns in time-varying functional magnetic resonance imaging (fMRI) data based on topological analysis. The oxygenated blood flow measured by fMRI is widely used as an indicator of brain activity. The signal is, however, prone to noise from various sources. Random brain activity, physiological noise, and noise from the scanner can reach a strength comparable to the signal itself. Thus, extracting the underlying signal is a challenging process typically approached by applying statistical methods. The goal of this work is to investigate the possibilities of recovering information from the signal using topological feature vectors directly based on the raw signal without medical domain priors. We utilize merge trees to define a robust feature vector capturing key features within a time step of fMRI data. We demonstrate how such a concise feature vector representation can be utilized for exploring the temporal development of brain activations, connectivity between these activations, and their relation to cognitive tasks.