Towards Benchmark Data Generation for Feature Tracking in Scalar Fields
Emma Nilsson, Jonas Lukasczyk, Talha Bin Masood, Christoph Garth, Ingrid Hotz
View presentation:2022-10-17T21:41:00ZGMT-0600Change your timezone on the schedule page
2022-10-17T21:41:00Z
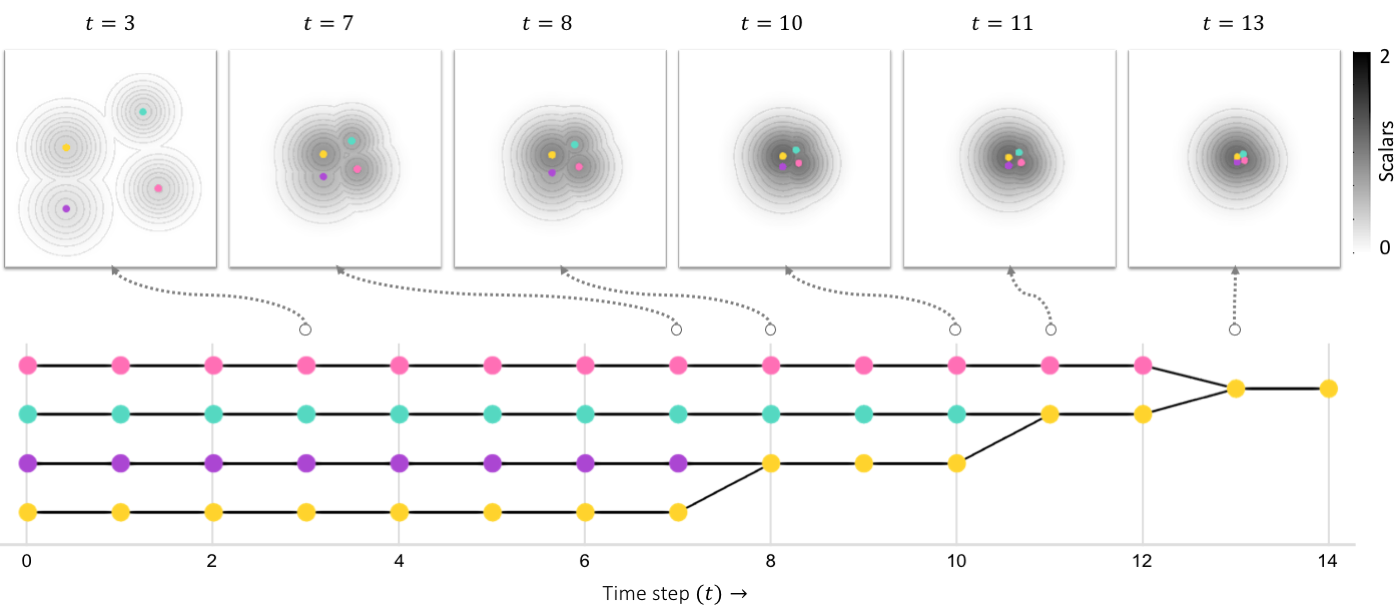
The live footage of the talk, including the Q&A, can be viewed on the session page, TopoInVis: Session 2, Early Career Lightning Talks + Best Paper Awards .
Keywords
Human-centered computing—Visualization—Visualization design and evaluation methods; Human-centered computing—Visualization—Visualization application domains—Scientific visualization
Abstract
We describe a benchmark data generator for tracking methods for two- and three-dimensional time-dependent scalar fields. More and more topology-based tracking methods are presented in the visualization community, but the validation and evaluation of the tracking results are currently limited to qualitative visual approaches. We present a pipeline for creating different ground truth features that support evaluating tracking methods based on quantitative measures. In short, our approach randomly simulates a temporal point cloud with birth, death, split, merge, and continuation events, where the points are then used to derive a scalar field whose topological features correspond to the points. These scalar fields can be used as the input for different tracking methods, where the computed tracks can be compared against the ground truth feature evolution. This approach facilitates directly comparing the results of different tracking methods, independent of the initial feature characterization.