How Do Algorithmic Fairness Metrics Align with Human Judgement? A Mixed-Initiative System for Contextualized Fairness Assessment
Rares Constantin, Moritz Dück, Anton Alexandrov, Patrik Matosevic, Daphna Keidar, Mennatallah El-Assady
View presentation:2022-10-16T14:24:00ZGMT-0600Change your timezone on the schedule page
2022-10-16T14:24:00Z
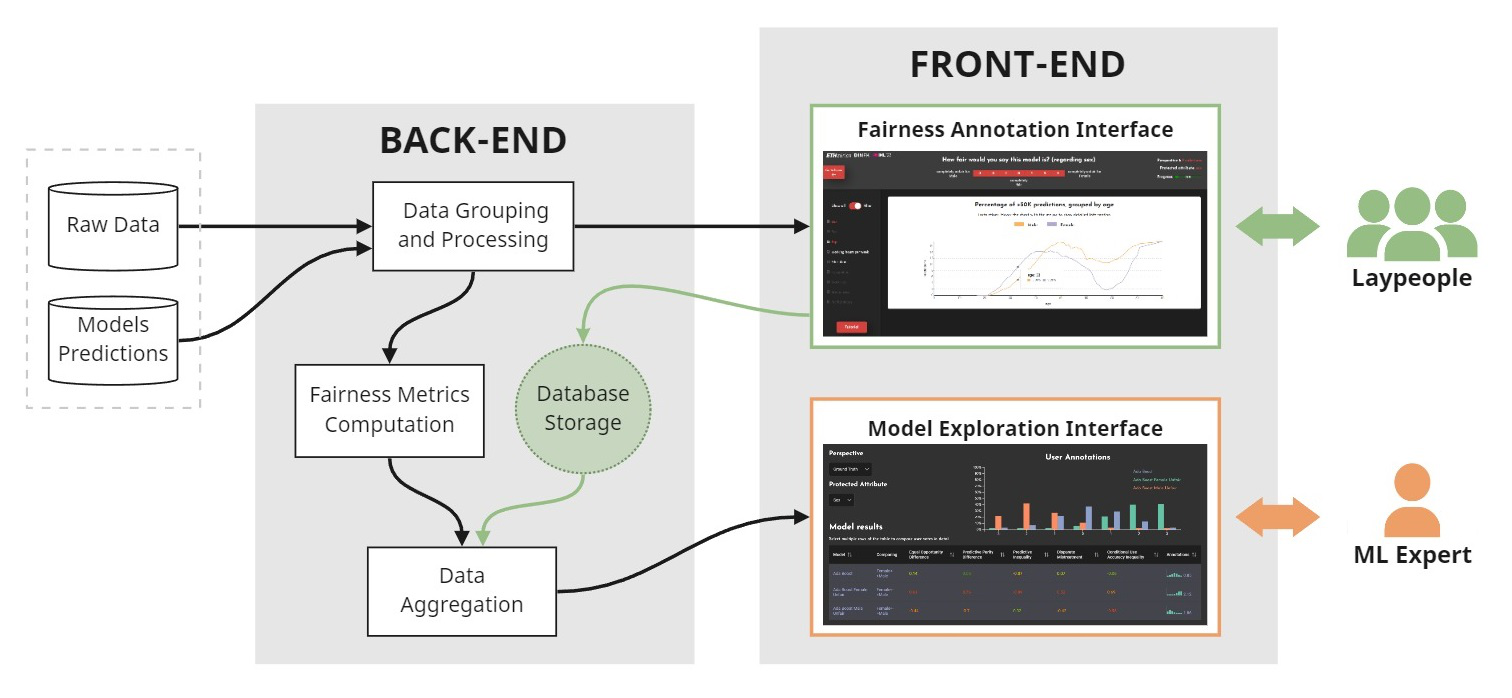
The live footage of the talk, including the Q&A, can be viewed on the session page, TREX: Session 1.