Outcome-Explorer: A Causality Guided Interactive Visual Interface for Interpretable Algorithmic Decision Making
Hoque, Md Naimul,Mueller, Klaus
View presentation:2022-10-19T14:24:00ZGMT-0600Change your timezone on the schedule page
2022-10-19T14:24:00Z
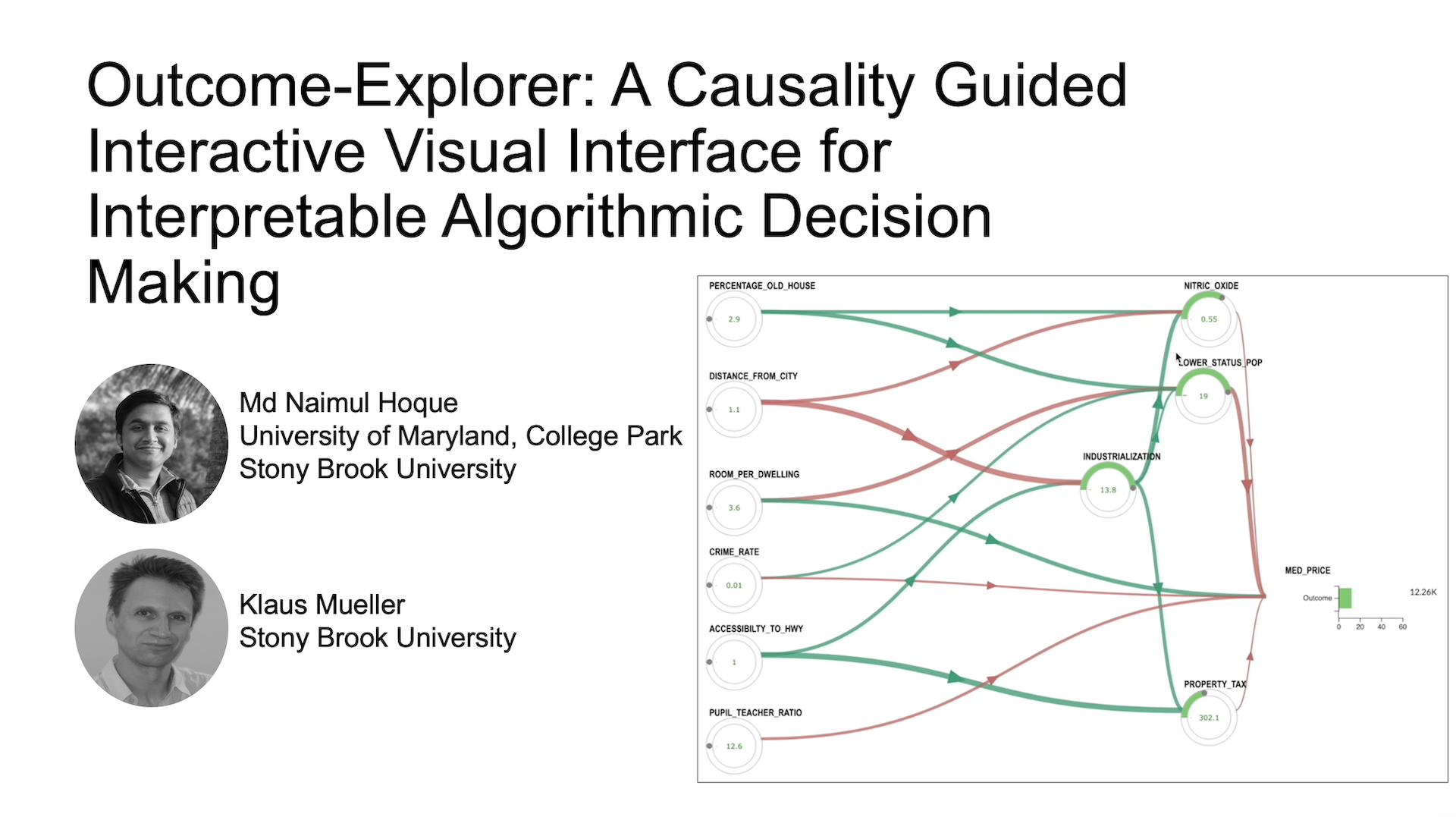
Prerecorded Talk
The live footage of the talk, including the Q&A, can be viewed on the session page, Decision Making and Reasoning.
Fast forward
Keywords
Explainable AI, Causality, Visual Analytics, Human-Computer Interaction.
Abstract
The widespread adoption of algorithmic decision-making systems has brought about the necessity to interpret the reasoning behind these decisions. The majority of these systems are complex black box models, and auxiliary models are often used to approximate and then explain their behavior. However, recent research suggests that such explanations are not overly accessible to lay users with no specific expertise in machine learning and this can lead to an incorrect interpretation of the underlying model. In this paper, we show that a predictive and interactive model based on causality is inherently interpretable, does not require any auxiliary model, and allows both expert and non-expert users to understand the model comprehensively. To demonstrate our method we developed Outcome Explorer, a causality guided interactive interface, and evaluated it by conducting think-aloud sessions with three expert users and a user study with 18 non-expert users. All three expert users found our tool to be comprehensive in supporting their explanation needs while the non-expert users were able to understand the inner workings of a model easily.