Combining Real-World Constraints on User Behavior with Deep Neural Networks for Virtual Reality (VR) Biometrics
Robert Miller, Natasha Kholgade Banerjee, Sean Banerjee
View presentation:2022-10-20T19:12:00ZGMT-0600Change your timezone on the schedule page
2022-10-20T19:12:00Z
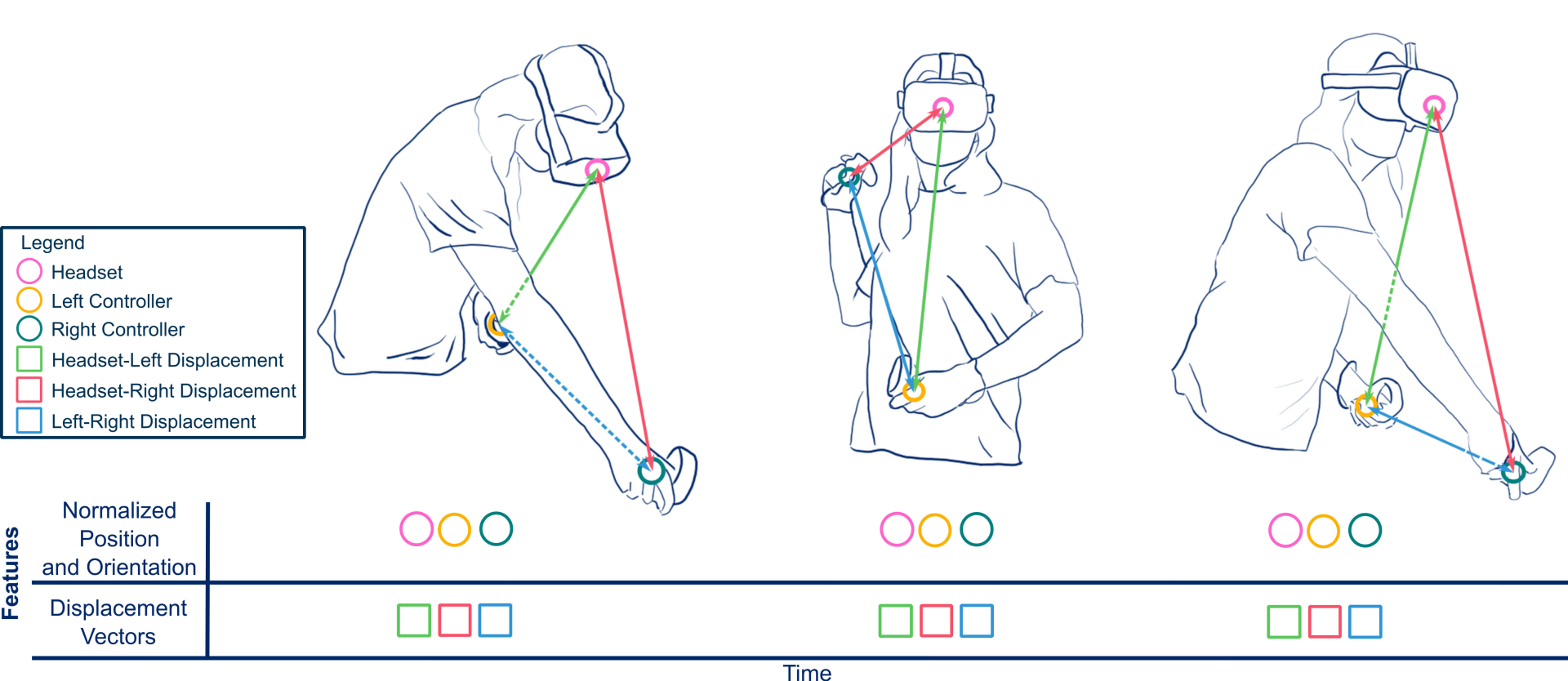
Prerecorded Talk
The live footage of the talk, including the Q&A, can be viewed on the session page, VR Invited Talks.
Keywords
Virtual reality; Biometrics
Abstract
Deep networks have demonstrated enormous potential for identification and authentication using behavioral biometrics in virtual reality (VR). However, existing VR behavioral biometrics datasets have small sample sizes which can make it challenging for deep networks to automatically learn features that characterize real-world user behavior and that may enable high success, e.g., high-level spatial relationships between headset and hand controller devices and underlying smoothness of trajectories despite noise. We provide an approach to perform behavioral biometrics using deep networks while incorporating spatial and smoothing constraints on input data to represent real-world behavior. We represent the input data to neural networks as a combination of scale- and translation-invariant device-centric position and orientation features, and displacement vectors representing spatial relationships between device pairs. We assess identification and authentication by including spatial relationships and by performing Gaussian smoothing of the position features. We evaluate our approach against baseline methods that use the raw data directly and that perform a global normalization of the data. By using displacement vectors, our work shows higher success over baseline methods in 36 out of 42 cases of analysis done by varying user sets and pairings of VR systems and sessions.